#231 Data Analytics and AI in Healthcare: A Conversation with Flatiron Health's CTO Catherine Miller
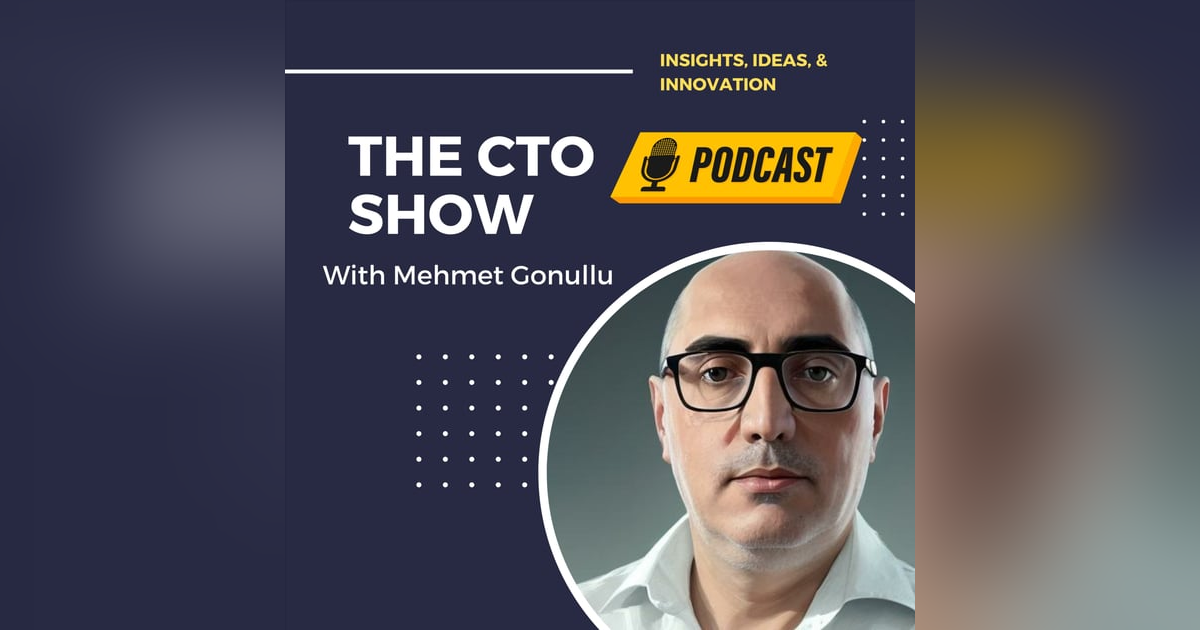
What if we told you that data could revolutionize healthcare, and in particular, the fight against cancer? You can discover the power of data in our enlightening conversation with Catherine Miller, CTO of Flatiron Health, a seasoned tech expert who transitioned into the healthcare sector. We dive into the profound impact of data analytics and how it can extend lives, inspire drug development, and provide a comprehensive understanding of treatment effects across diverse populations.
The second part of our discussion takes us into the captivating world of AI and machine learning within healthcare. Catherine elucidates how these technologies can decode the vast amounts of unstructured data from electronic health records and aids in extracting critical patient information. She also voices her concerns about the potential risks of AI, underlining the importance of multiple checks on AI models to ensure patient safety. Together, we dissect the pressing issue of data security, a necessity in our current digital age.
Lastly, we grapple with the persistent challenges of efficiency and innovation in healthcare. Catherine offers a wealth of insights into the hurdles faced by clinical trials, including cost implications, time consumption, and potential errors. We also broach the subject of the complex relationship between pharmaceutical companies and health tech companies like Flatiron Health. If you're an entrepreneur trying to break into the healthcare sector, Catherine's practical advice and advocacy for a health equity prioritization lens when building models are indispensable. So, join us on this stimulating journey into the future of health tech.
About Catherine:
Cat Miller is no ordinary CTO. She's been instrumental in transforming the infrastructure of cancer care by harnessing the power of real-world data and incorporating AI into health data analytics. Despite initial skepticism about AI's potential in healthcare, she has led the successful integration of AI into Flatiron's operations, driving forward efficiency and quality of data extraction.
Cat predicts a future where 95% of Flatiron's data could be extracted through AI models, a vision aligned with Flatiron's mission to redefine the boundaries of health data analytics. Cat is also an advocate for the responsible use of AI. As generative AI becomes more widely available and used across the health care landscape, it’s critical that there are standards in place that define high quality data in order to ensure the relevance and reliability of AI’s findings.
https://www.linkedin.com/in/catherine-miller-0177142
0:00:01 - Mehmet
Hello and welcome back to a new episode of the CTO show with Mehmet. Today I'm very pleased joining me from New York, Kat Kat, she's the CTO of Lecheron Kat. The way I like to do it, I keep it to my guests to introduce themselves, because I believe no one can introduce someone else better than themselves. So the stage is yours.
0:00:20 - Cat
Yes, so my name is Kat Miller. I'm CTO of Flatiron Health and my career has been in predominantly health tech startups and startup technology.
0:00:30 - Mehmet
Thank you very much, kat, again, for being on the show. So maybe it's like a traditional question, but what have you brought you to the tech world to be a CTO and why, specifically, you have chosen the healthcare to be your specialty?
0:00:47 - Cat
Yeah Well, why tech? I think it's an interesting question and I don't know that I ever chose it. I think it chose me. So I went to MIT in undergrad and I think at the time 33 percent of the student population was doing computer science. So I did computer science because it was the thing that people were doing and I graduated and I wasn't like, oh yeah, I'm going to be a computer scientist or I'm going to be on a tech career. This is like the early 2000s, and so the world was a little bit different. It just kept sucking me in. My first job was technically an analyst and they were like, oh, you can code, great, you're going to do code for us. And it just continued like that, where I just kept getting sucked back in.
Why health tech? It was an expressed preference. I never introspected and said, oh, I love health tech. But every time it came time for me to find a new role or look for other opportunities, I was always drawn to the healthcare opportunities, and so that just became a theme. Over and over again it turned out like oh yeah, I actually really care about healthcare and I think it's also fascinating. I find everything from the economics to the biology of it really interesting.
0:02:02 - Mehmet
Yeah, that's quite interesting and actually I'm a little biased because I have technical background. So already technical people, usually they do a lot of job that people they don't see, and when you do this in another sector like healthcare, which also helps people usually with their health, which is the most important thing in life, so I think this adds a double kind of a service over there. Now, when we talk about technology in healthcare, which is health tech, basically there's a lot of things that goes in. But I know, kat for a fact with your current work at your current company, so you focus a lot on the data analytics. Can you tell me more what exactly at Flatter on Udo and what kind of solutions you offer and why data is very important in healthcare in general as well?
0:03:04 - Cat
Yeah, for sure. So I'll start by just saying the mission of Flatter on Udo is to improve and extend lives by learning from the experience of ever cancer patient. So historically, what that's meant is that we use data generated at the point of care, so data that comes from routine care of patients, and we take that and we say, okay, if we could learn from every single patient with cancer, what insights could be developed in the world. So only 5% of patients are on clinical trials, so everyone else is a story that's lost to history unless you capture it in some way. So the fundamental idea was, hey, there's all this data out there, there's so much we could learn from it. Let's capture it and make it usable. And that's the history of the company. And we're now in a phase where we're expanding that.
So historically, we've been in the US, we're expanding internationally and we're also saying, hey, we have these pieces of infrastructure. How do they apply to things like clinical research or how can we expand point of care solutions? So that's the top level what we are as a company. But I can give you some maybe better examples of why you should care. I think maybe the idea of, okay, we could learn from these patients resonates, but people. Often the next question is like, okay, but how? And so this real-world data, this data that's generated from patient experiences.
One thing that's really interesting for me, having been in this for almost 10 years now, is the amount of blindness that we used to have to how many patients are diagnosed at a certain stage of cancer, or even how many patients are treated with a particular drug, or all these things that were very fundamental to understanding the cancer patient population were things that 10 years ago we really didn't have a great handle on. So what these things let you do is speed drug development everything from what patients are where underserved populations that we should be targeting to. How do I design a clinical trial that's actually going to recruit and be successful? So and successful in this case I don't even mean whether the molecule is good or not a trial that is able to reach endpoints and read out properly. So 80% of clinical trials don't recruit on time, so they have some timeline that gets blown out of the water.
And every time that happens, that's a drug that isn't available and that's cost that goes into that trial that isn't going to developing other drugs. So there are all these micro ways along the way of drug development that patients get lost or that things get more expensive. And to add to that, I think maybe something that resonates and is really easy to understand is understanding how treatments work in diverse populations. Clinical trials are younger, they're male, they're white, and so if you want to know how does this drug do amongst other populations, amongst women, amongst people of color, real world data and this data capture is one of the best ways to do it.
0:06:07 - Mehmet
Great. I think it's very, I would say simple explanation. I got it, although I'm not into health tech, so that was very self-explanatory, I would say. Now, because you were mentioning about collecting data and having these data sets from the trials, clinical trials and so on, the question that comes to mind and I know that you had done some work on that which is leveraging machine learning and artificial intelligence to help you actually and here I need your help actually to explain to me more. What are the benefits of it? Because when we say data, if I have too much data, the first thing that comes to mind okay, I can use machine learning because I can do some analysis on this, but what are other use cases where AI and machine learning would be able to help?
0:07:00 - Cat
in this case, yeah, well, I can kind of define the problem, which is especially in cancer. Our starting point is an electronic health record, so this is the way that a physician interacts with their patient and the way they keep track of their data, and so some things are stored in structured fields, so you have things like gender and age. These are things that are stored in a structured way, but then there is this huge mass of text and information that's stored in an entirely unstructured way.
The physician sits down and says Mrs Smith, isa, very lovely, blah, blah, blah, and then they tell you all the things about that cancer Also, I should maybe take an opportunity to mention when we say cancer, cancer is hundreds of different diseases. They all kind of share this idea of, like you know, a cell that is dividing too fast, but they are wildly different and the things that we care about in terms of understanding and treating them are also wildly different. So what you have is a big blob of text. You have, you know, many, many documents, and so if you want to understand and you want to do any kind of analysis or research on that data, the first thing you have to do is get that information out of it.
You have to do some sort of text extraction, and maybe this is feeling more natural to people in a world of LLMs, but it's actually a hard problem. Some pieces of it are really easy. So smoking status is a very easy thing to extract because it's sort of always in these like very easy to deal with chunks. Whereas, did the patient have a full resection of their disease? Did the patient have a progression of their disease? These are complex concepts that are much harder to pull out.
So, to answer your question like what is it even doing? A really key thing that it's doing is it's taking unstructured, unformed information text and turning it into actually valuable information that can be used, you know, for insights, and that's something that we've been doing for a long time in a sort of, you know, sort of careful and cautious way because, like I said, it works really well for some things, works not great for other things, and so that's been a process over the years as techniques get better, you know, as we have more labeled data of expanding the ways that we can use ML to extract that information from the data.
0:09:20 - Mehmet
Yeah, that's very clear to me now because you mentioned, you know, for people who are not familiar, when we say unstructured data, which is meets like data which is sitting fragmented all the place and you don't have the labels on it you mentioned, you know, like as a data sitting in a record right, and, as you to your point, there are some stuff which is easy to extract, like you know, gender, married, not married, single, I don't know, like all these, like binary options, let's call them, and fine, even like when it comes a little bit to more.
Let's say, if the MD writes there some, you know, someone comes to the clinic and they write some description okay, this lady came and she was suffering this, and then we described her, this drug to her, and so on. Now, this is all in the sense of text, which is, as you said, people think it's easy, but it's not. But out of curiosity here, what about the media that get generated, cat, like you know, the x-rays, the c scan, you know the all these data? Like how easy it is, or have have you tried, I'm not sure, like to see a way, because I think when you go there, usually from my knowledge is we rely on a physician, or I mean an MD, to tell us what they have seen and they write it in their reports. But is there any way that AI can leverage? You know, to use this media.
0:10:55 - Cat
I mean for sure. So this is an area we've been exploring for a couple years. There's actually some interesting things about the fact that those images are not always stored in the same place as the rest of the record. So you might have a medical record that contains all the text, but then there's a separate place that you know, for example, mris or PET scans are kept. So there is actually an interesting thing about like, where do you have data? So we spent quite a while getting access to more of that scan data and I would say one. It's an entirely different problem. So there are companies out there which I'm actually really excited about that are doing radiology assist reads and trying to use ML to augment physicians to make it, you know, radiology reads faster and better, and I think that's a really cool space. That is really about, I think, more machine vision, which is also AI, but a totally different sort of branch. So I think that's really cool and I'm really excited about the work that's being done there.
But for us, I think we then have a multimodal problem of we've got a scan and in some cases it might be useful to have the scan itself. In some cases, it might be useful to do a read on it and then compare it to what's in the physician record, or do a read on it and just make that information available. And so that's something that we have been doing over the past few years is to make that information available in sort of like a broader corpus. Another way you mentioned scans, but I'll just mention another way that we're bringing in extra data. Genomic data is another example, not necessarily of multimodal, but of something that is, you know, a very different kind of information stored in a different way that is potentially very interesting to certain use cases. And so, yeah, I think all of that together creates, creates more power, creates more knowledge in the world. If you can bring all those things together.
0:12:47 - Mehmet
Absolutely. Now, one thing which is kind of funny, because I had a guest the other day, sid Mohaseb, and we were discussing, and previously I had another guest, kasha, which is she's an MD herself. So people sometimes tend to be skeptical about AI. So when you tell them I'm using AI for something and I think this started because I would not say because of chat GPT, but I mean, you know chat GPT, you know it.
Let the mass people see what AI can do, because till some, you know last year, okay, people knows about the AI, but no one knew that you can actually have this large language model and you can do these analysis. And all of a sudden, all the people around the world they know, ah, there's this AI thing that can do this. And people start to talk about hallucination of AI. And yeah, this AI doesn't understand anything and it's a dumb from of course. Now we're talking science, we're talking technology. What you can tell you know people who might have heard it. I would not. I don't want to blame them directly. Maybe they have heard it from their friends, they've seen it on an article somewhere on the internet. I want you to explain why it should not be a concern and actually to the opposite. It should, it could be very beneficial.
0:14:05 - Cat
Well, I mean honestly, I'm I've been an AI skeptic too. Flatiron was built on having humans do a lot of this work, so I've talked maybe more about the technology of text extraction, but it's built on the back of having a lot of humans going in and labeling data and doing a lot of the harder problems. And so, interestingly, like we're a company that was built on the idea of 10 years ago, ml cannot do this. Like you think, ml can do this, but it cannot, and I think that was very accurate. And it's not just large language models, it's actually a lot of the developments of recent years. It's deep learning at some of the techniques that have come along. I think that the ML of today, you know, it's not. It's not the ML of when I was in school, it's not the ML of 10 years ago. It has different capabilities. None of that means that that it's perfect or magic.
I think it's closer to magic than it used to be. I think you know, five years ago, even two years ago, I feel like you would use the word AI and someone and whatever people thought it could do whatever they dreamed of. And it can't necessarily and I think we're large language models bridge a gap that we had in terms of being able to have a single model that is flexible and can do many things. So that's all background. I will actually answer your question, which is like why shouldn't people be scared, or or why is it okay? I mean, it still can be used really badly there are.
What I think you need to ask is how are you dealing with the possibility of hallucination if you're using a large language model or if you're using a more traditional model? Where do you put your cutoffs? How do you think about where, what levels you're willing to trust? How do you think about, maybe, layers of checks right, so you can use, you know, llms at various points to not only generate information, but also to check that information along the way? So, like it's not that there's no risk, I think there's also risk in people, by the way, like we've had humans doing this for a long time, and humans are flexible and you can teach them lots of things and you know there's a lot of value to them, but they make mistakes, and they make different kinds of mistakes than computers do. So actually, one of the things that we found incredibly impactful is to combine the two.
So maybe you use your, your large language model to do a summarization of a document or to tell you what part of a document is gonna be most relevant, and then you have a human pull that out. Or maybe you have a model guess or predict what something's going to be, extract what something's going to be, and you have a human check it, and maybe you only do that a certain percentage of the time, because that's how you're checking your model. There's a lot of different ways to do it. What I do think is probably still not in the realm of success is oh great, I've got a patient record, I've got a large language model, boom, I've got answers. I think that's still, you know. I think to say that that's possible, I think, really undervalues the amount of engineering and thought that has to go into ensuring that you get the right answer.
0:17:04 - Mehmet
Yeah, 100% Like as any other technology. You know, if it falls in the hands of wrong people, it can be harmful. So, yeah, this is the nature of the life we live, so nothing we can do much about it. But I encourage to try to protect this technology and try to harden it from falling in the wrong hands. And this will lead to the question because you know one of the concerns usually, because we know these AI models, whether the traditional one or the new ones, they are actually collections of data. And you just mentioned and here we're talking about healthcare, so we're talking about real clinical data so how important it is to, while doing all this, keeping the privacy, keeping you know the data secure, right. So, and you know, as a CTY, I think for you it's like a big responsibility as well. I know like you might have a CISO as well there, but I mean as a CTO because you are responsible for you know the infrastructure over there.
0:18:12 - Cat
Yeah, I mean, it's been the thing we think about, the thing that keeps us up at night since day one. You know, maybe to take a little bit of a as someone who studied economics, I always like when incentives align, and so I guess the good news is that if we ever we're not good custodians of data, if we ever had a major breach, I think that would be the end of us as a company. So we are very incentivized to make sure that we are keeping data private, and I think a question that we often ask. Obviously, when we deliver data, it's anonymized. We strip out all identifiers. You know we have an expert to certify the de-identification of data before we deliver it, so that's obviously a huge part of it.
We would never give someone's identified information out in any way, but I think it's all you're also referring to like okay, but you have all this data stored somewhere, you have an infrastructure. You have to keep it safe along the way. Absolutely true and honestly, like one of the most important things and one of the things that kind of pervades everything we do is could this hurt a patient? Either because we let patient data out into the wild, which obviously would be a problem, but also as we make micro decisions. If this data was wrong, if this ML model was wrong, how would that hurt a patient and how can we test for that and avoid that? So I think and I'm sure we're not the only healthcare company, healthcare tech company, that has this as like a North Star, but like, do you know, harm to patients is absolutely like the first and most important thing.
0:19:56 - Mehmet
Yeah, 100%. Now, one thing I get to know that you predict that, by like 95% of the data that you I mean you try today to extract, would be, I mean, extracted through AI models. So what are the key milestones to achieve that, to achieve that result, Wow.
0:20:21 - Cat
I mean I've definitely been saying I think we can get to 50% by the end of next year. Key milestones along the way. I mean some of it's. I think what's interesting about large language models if you're doing everything with traditional ML, you're building a model for every single thing that you need to extract. So we have really large data models. They cover things all the way from stage of cancer to things like the depth of the resection in a melanoma side of colon and cancer biomarkers, progression of disease, all these things that are.
It's a really broad data model and, as I mentioned, cancer is not one thing. Cancer is 100 different diseases. So if you have to build a custom model for every single one of those things from scratch, you will spend a very long time getting to that 95% and you'll honestly never get there, because there's always new things. There's this is a good thing there's always evolution in practice. There's always new things on the market.
So I think the key milestones are really finding ways to build one thing and have that applied to a large swath of the data, whether that's a single model, whether that's an LLM strategy, even whether that's, like I kind of mentioned, a hybrid strategy of this is a model that we can apply broadly and it's not as good as a custom model, but then we use people on the other end and that's actually like the fastest, best way. I think this idea of how do you, how do you find things that apply more broadly, how do you find ML that doesn't require months of sort of careful crafting to get to you to the perfect model, that's actually like the milestone, and having those along the way, maybe for different chunks of the data model, to me is where we need to get to and the way we'll get to success.
0:22:20 - Mehmet
Yeah, that's great to hear and I'm looking forward. So, like you mentioned at the beginning also, kat, like you're doing a lot of expansions now and I get to know also that you've doing some collaboration with like in the US, with the FDA, and you're trying to establish some partnership in Europe, in the UK. So my question is, when we talk about and you kept repeating this a couple of times about that cancer is not one disease, I want you, of course, like we are not into a medical show, but I mean in a simple term, from technology perspective, why you highlighted this and underlined this. And because I know this is, I'm asking because that means you need to deal with a lot of data sources and now that you are working on a global level, so that means you will have more broad data sets that would come to you.
0:23:24 - Cat
So, if you can just touch on this, so for people who can be enlightened on what we are talking about, yeah, I mean I think the reason I highlight this is a couple of things that the fact that cancer is not one disease, I think importantly, as you said, it means that we're not developing one data set. We're developing dozens or hundreds, depending on what we're talking about and, for example, I have worked in other diseases. I've worked in, for example, diabetes in the past. Diabetes, by contrast, is a very simple disease. There's two versions of diabetes and, yes, there are certain subtypes under them, but they're not treated radically differently. There's a set of drugs you give in a certain order, there's a set of tests you order, and even though the underlying causes for even different type one diabetics might be different, the way you treat the disease is substantially similar and the information you need to treat the disease is substantially similar In cancer.
So maybe the easiest way to think about this is something like a lung cancer, which is a solid mass inside your lung, versus a blood cancer, which is that you have certain types of cells that are mutating and circulating throughout your body. I think it's very obvious that one you can potentially cut out and another you cannot, and that's such a wildly different disease. So I think that's really helpful to understand, when you're thinking about things like building out data to support different diseases, that they're not all the same, that they do require different information. It's important when you think about where cancer money is going and the fact that getting a better treatment for breast cancer doesn't necessarily mean a better treatment for prostate cancer or hepatic cancer or any of those right. So I think, as a consumer, that's an interesting and important point to understand. Also, different cancers have wildly different survival characteristics.
These are all things that I think is a layman, certainly things I didn't really comprehend before I joined Flattern, and maybe another interesting fact about it is as a doctor, as a cancer doc. There are specialists in particular cancers but a lot of especially community practice docs are dealing with all of them. They are, all you know, all-comer cancer docs and that is a really hard problem to be able to treat all of these different diseases effectively, to keep up with the literature on all of them. And so, again, as a technologist, as we think about problems we're solving, and as a company that has solutions at the point of care, I think that's another thing to think about is how do we support docs who are treating, you know again, not one disease, but hundreds of diseases, some of which are really common. I'm sure they see. You know every doc sees a lot of breast cancer patients. You know a lot of docs are not necessarily seeing the rare tumors that are out there.
0:26:13 - Mehmet
Yeah, got it Now. Also, I got to know that you started a collaboration with Sanofi to do some work on having you know the clinical trials more efficient by streamlining the data acquisition at the point of care. So why this is important, Like, tell me more about that.
0:26:36 - Cat
Okay. Well, clinical trials are, I think, a holy grail in some ways, because if you come into it from a technology or an engineering perspective and you look at what we do, honestly it looks a little absurd, like the cost of it, the amount of pieces that are built up around it. You would never build that system from scratch. Like this is a system that's evolved over, you know, 50 years and it's a very conservative system for a great reason right Again like let's not harm patients. But what that often means is that you can't make changes or you do things the way they were done, and so we have systems that reflect a 1970s level of technology and interconnectivity that are not serving us in a modern world. And the reason you should care is that clinical trials are incredibly expensive. They're a billion dollars potentially, depending on the size, and so the billion dollars. You may not care about the pharma company losing a billion dollars, but what you should care about is that it's a billion dollars that is not going to other drug development and also it takes a long time. So you might have a molecule that is ready to hit a phase one trial today and it could be easily six or seven years before it gets through its phase one, its phase two, its phase three, and even then it might have more questions that need to be answered by a phase four, and so that is a length of time in which patients are not getting that treatment. It's important, right? I'm not saying that clinical trials are not there critical. We need to use them to understand whether that treatment is in fact valuable in any way. But you as a consumer should care about efficacy and efficiency of clinical trials because of the weight that they put on the technology, ecosystem or the infrastructure of healthcare.
And so I think that there is a number of ways and you know I'm not alone in this.
I think the market also thinks there's a lot of ways in which we can improve clinical trials. One of the sort of more obvious ones is the way in which data is entered. A physician who's participating in a clinical trial records all their information in their electronic health record like they do normally, and then they have to copy it over into the database for the trial, and that has a lot of problems. One, it takes time and energy, and often you have to have like a separate person to do that work, and it leads to potential errors. Right, so you know, human copying is not a perfect science and so, just as, like a starter point, like that's the very beginning of it, you can see a real opportunity to directly connect the data that the physicians using in the clinical practice with the data that ends up being sort of input into the trial. And like I think that that the more you look at clinical trials, the more you see that there's opportunities to make them smoother and more efficient.
0:29:33 - Mehmet
Great explanation. Even you know I'm not familiar with the topic, but you explained very well. Thank you, cat, for this. Now you know from again, because, being a CTO and coming back to the CTO, so you need so your customers usually are, you know clinics, right, and I mean drug companies. Maybe am I correct. Yeah, I'm curious to know because you know and this is the CTO show, and being in a company and having the role of CTO, whether you are still start up or growing up, it's important to understand the customer. So how, how, how say different, or how is it harder to deal with? You know this persona which are, you know, like healthcare people versus maybe you know having other Personas in different industries.
0:30:29 - Cat
Well, I think the thing that's hard about healthcare so you mentioned that that our customers are both the practices, so the docs and everyone else who works at the point of care helping patients and also, on the flip side, the pharmaceutical companies who are using the data to make their you know processes better, faster, stronger. So it's two, two pretty different worlds, you know, I think that working at the point of care is hard because the physicians at the point of care are so overwhelmed, underwater I don't know what the right word to use is but they're under such strain. They have to go through patients incredibly quickly. They are facing sort of decreasing revenues every year, as, as sort of the systems in, this is a US specific thing. I would say, though, I don't think that US is the only place where doctors are struggling and they're feeling like their tools are not serving them. Right there, they're feeling like they're spending less time with patients. They're more, more time entering things, and so they, very reasonably, are not that they're interested in innovation at like, a conceptual level, because they want things to get better, but at the individual level, every time we move a button on them. That's something that they have to relearn. That slows them down.
So I do think that innovating in that space can be hard because the users can afford so little in terms of change cost because of the strain that they're having.
So I think it's a place where you need to have a ton of empathy for those individuals.
We obviously have a lot of cancer docs on staff who live in that world, who still have clinical practices, and I think that's really important because, versus you know making a, you know a lot of people who are in the center of the site. They can maybe test things all day long because you know if I need to go by toilet paper, they're not super worried about that. You know taking me an extra one second, and so I think we're, I think cautious and sure of the changes we're making, that they're actually genuinely an improvement. We can't do things like a B testing because it messes with them so badly. So it's not I don't know what's if it's even that. It's the healthcare part that's hard, although certainly there are regulations and it's very costly to develop in the case. That is part of it. But I think just anytime you're building for a population that has so little slack in their lives, I think that that is is hard, and this is the tool that they're using every single day.
0:32:59 - Mehmet
Yeah, please, please go ahead.
0:33:01 - Cat
Well, and I think on the farmer side, I think that that's much more like a normal B2B relationship. You know, pharma companies are made of people. They're trying to get their stuff done. They're trying to figure out if, if there's a faster way or if our data can help them. That they're. You know, every industry has its own characteristics, but in some ways that feels like a much more normal relationship that I think folks who have been in B2B spaces probably are.
You know, it wouldn't feel wildly different to them though that you know the structure and the politics of every organization is its own, unique. You know special snowflake.
0:33:33 - Mehmet
Yeah, I've heard it like from multiple people who are in the healthcare. So, you know, and I think it's a common trait, is the regulation that you mentioned, because you know you cannot move as fast as like maybe other industries, and it's not only in the US. In the US you have the FDA, in Europe, like, there's like something similar here in Dubai we have something very similar also as well, and you know to take approval sometime it take too much time. It was like their number one. I would say it's not struggle, but the kind of a challenge you know to move things forward. So, yeah, and then it's coming everywhere. Now we talked a lot about you know ML and AI, so, but what other you know, like emerging techs are you seeing having big impact in the healthcare industry, in the tech, of course?
0:34:28 - Cat
Well, I think a lot of it does come back to AI and ML in some ways, in the sense that, where I think that there's a lot of opportunity, and in the healthcare industry in general, is in the glue.
So there's, you know, things that touch docs and I just talked about how hard that can be because they have sort of limited resources, you know and things that touch patients. And then there's you know, obviously, the other end, sort of drug development. There's a whole bunch of little interstitial systems in between. Think about, like, medical coding in the United States is a career that people can have. It is a thing you do, which is you look at a record and you say this is how much we should charge for it. Insurance processing is another one.
I think that there's and I think that that will see, a lot of companies in these like very specific spaces of I can make this pipeline, this particular part of the problem, better, and I think that AI is one of the tools that's actually going to be critical and that I think that the ability to sort of quickly process data, especially in cases where the cost of being wrong isn't quite so high so you know, being being wrong.
Telling a doctor, oh, we think your patient has stage four lung cancer, and if you're wrong, that's like that I mean, presumably the doc will catch it, but that that's not great, right? The telling an insurance company we think that this is a level three visit and it's actually a level two visit costs money but maybe doesn't have the same implication on human lives and it's something you can ask it about as an economic aggregate. Is it okay to have a certain level of error here? And so I think that there are pretty unsexy but interesting parts of the healthcare ecosystem. Even when I talked about with clinical trials, you know, it is sort of like how do we remove data? Entry is one piece of a larger puzzle, and I think if you looked at the entire healthcare ecosystem, from drug development all the way to patient care, there are these little little bits that can be, that can be carved out and and improvement made on them.
0:36:33 - Mehmet
Mm, hmm. So if I want to ask you, because coming back and I agree with you, like it's the AI and ML that that gonna play a muscle of the role in getting things to advance stages, if we come back, let's say, and record the same episode after five years or 10 years would be talking about AI doing 95% of the job that it's done today by humans. Do you think so?
0:37:02 - Cat
Well, what do we mean by the job? I guess you know is a higher course not.
0:37:08 - Mehmet
Not replacing doctors. You know, I know that the hiccups there, but at least you know from, let's say, drug. You know getting a new drug formula, let's say producing a new drug.
0:37:22 - Cat
Well, I think that there are a ton of places where it's speeding it up right, like something that isn't even in in Flattern's wheelhouse. But protein folding and the ability to try out a bunch of different drug models in CTU is like a really cool Use of technology that's been going on for a while. Of like can we, instead of sort of hand crafting a couple of molecules, can we have an automatically generated 2000 molecules and then an algorithm that helps us pick which ones are plausible, like that's been going on for a while? I think that I mean it's like. I think it's technically no, I don't think it'll be 95%, but I think that it will feel like a lot of jobs have changed dramatically.
I'm sure you've talked about on the show, you know, the job of engineering changing and some of and I think what's interesting about it is I think some of it will go unnoticed. You know, even in my answer to your question I was like yeah, aiml, and I completely glossed over the fact that that data technology has come so far in the last 10 years that we're using completely different data platforms, which is crucial in being able to have large data sets that have heavy processing. But I glossed over it almost because I like forgot that it didn't used to be that way, that we didn't used to have easy access to, you know, like things that are like like spark underlying them or easy ways to have data live in a bunch of different places at the same time. You know, that's an interesting thing to think about is, I don't know that in 10 years we will even kind of realize how much it's changed, because it will be a new normal for us, and so I think there are some jobs that will go away.
I think there's a lot of things that will change, I think, for physicians. I hope that they change because I hope that the job becomes more. One of you know the computer is better at taking its own records. It's better at sort of knowing what you're likely to want, to suggest something and then you, as a physician, spending time with a patient, understanding the patient's priorities and selecting among a couple of curated options and in some cases, saying you know, no, you're wrong, we need to do something else. You know that's what I'd love the job of a physician to become or to get back to in some ways, but I think it'll happen in incremental pieces and by the time we get there, in the same way that I think we have forgotten what it was like 20 years ago. I think that that will be our experience.
0:39:47 - Mehmet
Yeah, 100%. And what we repeated here on the show Kat, is it's kind of augmented ability to the people, like because, yeah, it will not replace a doctor, but it will replace a doctor who doesn't know how to use AI, right, so this is what we. For example, we said it will not replace an engineer, but it will replace an engineer who doesn't know how to use AI. It will not replace even office people I mean people who works at the office but if they don't have the ability to leverage this technology, they will struggle and personally, I don't think AI will take over jobs, actually to create new kind of jobs Like.
This is my theory and we might be doing something completely different, but look like there's a fact people like yourself will always be needed, because actually you need to be the brain behind it, For example, your team behind you also as well and this is coming from a technology background. You know I'm biased again.
There's a lot of things that goes in the background that people doesn't see, so and excuse me, Kat, because I'm taking this part from you because, and especially in healthcare, because I had the privilege to consult for some healthcare you know institutes before when I was in the corporate job, and you think, yeah, like they are just a hospital and you know like they are sitting behind computers but there's a huge systems behind there. You know, and you know the clinical systems, you know patient management systems and all this. It's not something easy, guys. And you know, kat, like really I always appreciate people like yourself, especially because now you are working in something much bigger that you know you have a lot of things you have to deal with. And saying this, I gotta ask you a question. Did I miss to ask you anything?
0:41:36 - Cat
No, I think we covered quite a wide range here.
0:41:40 - Mehmet
Yeah, so this is my famous end of the show question.
0:41:45 - Cat
Maybe actually let's talk a little bit about we touched on a little bit, but like bias in healthcare, because you know we talked a little bit about what should people be scared of with hallucination. But I think you know, bias is obviously something that comes up kind of every time you talk about AI and healthcare.
And it's very real right, like we've seen examples, we know that models reflect the data that they're created with, which is the world we live in, which is a very unfair world with a lot of disparities, and so I wanna like acknowledge that and say that you know, first and foremost, I think that everyone working in this space has to be using, like a health equity prioritization lens when they're thinking about their models, when they're thinking about testing their models. So, you know, I have a model. The first thing I'm gonna do is look at how does it treat patients of color? How does it treat, you know, women Basically like? How does it treat these groups that are less represented in the data or have historically been sort of discriminated against in various ways?
I also think there's a huge opportunity, because the same place that an algorithm can amplify bias is also a place where an algorithm can be used to dampen bias. So what I can't do is go talk to every single physician on the planet and talk through the decisions they make and try to figure out why, you know, their black patients are getting this prescription a little bit less. You know, what I can do is build an algorithm, test it thoroughly that, you know, has more equity or helps to correct some of that inequity, and I think that's a huge opportunity that we need to be as excited about as we are scared of the bias inherent or the risks that could come along with it.
0:43:34 - Mehmet
Mm-hmm. I just remembered one question that I should have asked you, kat, and it's kind of also important as well. You know, with the experience you have with healthcare, you know, and over the years Now, let's say, do you advise, you know, fellow entrepreneurs, and, of course, like techie people, to go into healthcare, and if they decide to, what are the advice that you would give them?
0:44:03 - Cat
I think, healthcare is really rewarding and it's really hard. So we talked a little bit about it's slower to develop, both because of regulations, but also because you have to be careful and also because a lot of the individuals are under a lot of strain. So you know it's a high impact potential. You have to have a really good plan, so it's not a place where you can sort of flounder around and say, you know, as someone who's never been in it, oh, I think this is a thing that will work. You have to have a lot of input and a lot of help from people who are in the industry. This is probably true of any industry, but I think it's so especially true of healthcare that you need to have physicians and scientists who are in it helping you along the way and be prepared for it to be a slower road than you know.
Oh, I made a social networking platform or oh, I'm selling a new thing. It's a different path and we've experienced. I think we've had a lot of success and we've also experienced setbacks in terms of how fast the world was going to move, how quickly the state of the world was going to change, how quickly things would be accepted, and that's a reality, I think also of being in the healthcare space, is that, for very reasonable reasons, the world is going to move slowly, slower on that than they are on you know, the next Candy Crush? What is that you hold to reference? Do we think about?
0:45:30 - Mehmet
that anymore? I don't know, yeah, like guys like don't think about healthcare startups like to be.
You know you will be jumping and drinking the bells very quickly and doing all this. It's like a long-term game. But, as you said, I agree with you, kat. I have a lot of friends who works, you know, not necessarily in startups, but I mean in the healthcare, in technology, and yeah, they tell me it's rewarding because every time they see, for example, even if they see a lot of people, for example, even if they are like working in the IT department, in the operations side, and they say, okay, like every time we know that because of the system that we have just installed, someone's life gets saved, we feel you know the reward over there, like it's something it's not about money all the time, it's also about you know the human touch as well, kat. Like I don't know, like did we miss anything? Any more things from your side, Please?
0:46:21 - Cat
I think we're good now. I think we did it.
0:46:23 - Mehmet
Okay, great, because I may try to make sure as much as possible. Oh, just, you know, I had to ask you something when people can find more about you and about the company.
0:46:34 - Cat
If you have a question, yes, so for Flatiron it's flatironcom and for me probably my LinkedIn. Kat Miller is my LinkedIn. I am not an active, a particularly active LinkedIn user, but anytime I have a speaking engagement or I'll certainly post this on there, and so that's where you can kind of find what I'm up to.
0:46:55 - Mehmet
Okay, great, I will make sure that I will put the links in the show notes. Kat, thank you very much for giving the time today. I know you have a busy schedule and you're building great things over there and for the audience. Guys like I hope that you find this useful. I find it very useful, honestly. Keep the feedbacks coming. We're trying to make a mix between all, I would say, the verticals across the technology. Even we are trying to bend in something not tech, necessarily marketing sales. So for you, if you are a young entrepreneur or startup founder, so you need to understand all the things going around. So I'm trying to, as much as possible, have this mix. And thank you for the feedbacks Like I'm getting them, I'm reading them and I need to see even some not only thank you, a great show Like. If there's something I need to enhance, please let me know. Guys Like I love to read these as well. So, thank you very much for tuning in and we'll meet again very soon. Thank you, bye, bye.
0:47:54 - Cat
Thank you.
Transcribed by https://podium.page