#246 Revolutionizing Patient Care with AI: A Conversation with Michael Armstrong
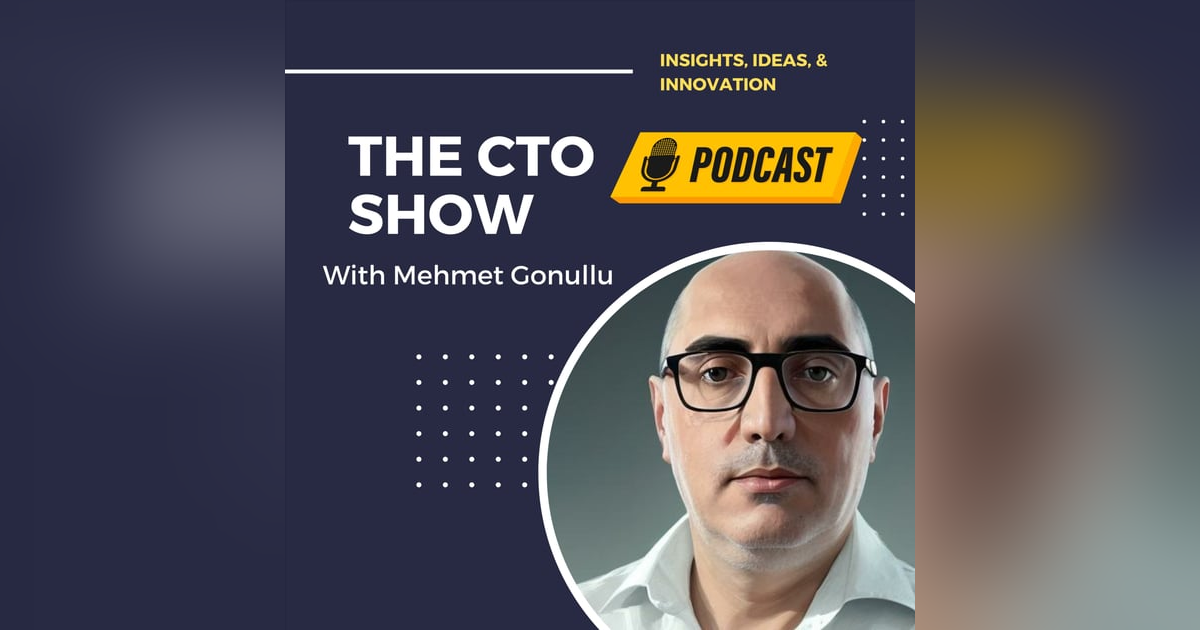
Ever wonder how AI could revolutionize the healthcare customer experience? We've got Michael Armstrong, the CTO of Authenticx, joining us today to shed light on this fascinating intersection of tech and healthcare. Michael paints a compelling picture of using AI to analyze interactions from call centers to glean unique patient insights and address customer issues. He also explains why traditional surveys are falling short and how AI can fill that gap.
We take a deep dive into the Eddy effect model and the safety events model, discovering how these AI models are used to measure friction, NPS and identify adverse events. Michael emphasizes the importance of relevant data sets in creating these models and how Authenticx ensures they're using the most pertinent data. We also explore how these models apply to call center conversations to foster better customer and patient experiences.
Lastly, we delve into the future of AI, exploring the potential of AI in job replacement, answering customer queries, and revolutionizing customer experiences. We also discuss the importance of encryption, the effort that goes into redacting PHI, and how a single conversation can serve multiple purposes. Join us as we unravel the complexities of training AI algorithms and the challenges and opportunities that come with implementing AI. You don't want to miss this insightful episode!
More about Michael and Authenticx:
https://www.linkedin.com/in/michael-armstrong-938a526
https://authenticx.com
0:00:01 - Mehmet
Hello and welcome back to a new episode of the CTO show with Mehmet. Today I'm very pleased to have with me Michael Armstrong. Michael, he's a CTO and I like to keep it for himself to introduce what he does and his company. So, Michael, thank you again for being on the show with me today. Can you please tell us a bit about yourself and what you do?
0:00:23 - Michael
Yeah, thanks for having me. I'm looking forward to it. So my name is Michael Armstrong. I'm the Chief Technology Officer at Authenticx. Well, it depends on who you ask whether you ask me or our CEO but we're in the customer experience space, so we really focus on customer and patient experience and it's really the intelligence, it's the insights that we can glean from conversations. So our philosophy is really like your patients or customers they're telling you what your problems are. They're telling you what you can improve. They're telling you where the friction is. You just got to listen to them. So that's really kind of the business focus that we take is really trying to understand this and helping clients solve these problems and understand this. Of course, we use a lot of AI because we do this at scale like massive, massive scale so of course, ai has to be a part of our strategy.
0:01:24 - Mehmet
Great, great. And thank you again, Michael, for being here today. So the way I like to do it and because we talk the same language. So, from a city perspective, as a company, and you as someone who works on the technology side of it, I'm sure that you spotted some problems right and you mentioned a couple of them, but what was really the pain that you tried to solve with Authentics and CXN? What triggered? Also, like you said right, this is something we need to fix because it's causing a lot of problems. So, if you can share this with us and elaborate more on it, yeah, definitely yeah.
0:02:12 - Michael
So what we saw? There's a couple of things. First off is healthcare. If you're familiar with healthcare in the US, it's expensive. The care is really it's been evolving in a way that's really not really beneficial to patients. So the care is decreasing, the costs are increasing, there's a lot of different people in the mix and it's just really complex, like it's a really complex problem. And at the same time, we also observed a lot of companies. They're putting focus and energy into customer experience and patient experience, but they don't really have a great way to sort of understand the problem set.
There's a lot of surveys, right. I'm sure you've seen a lot of surveys things like NPS, right? Net promoter score is a very common thing we see all the time, right, and we looked at that. And of course, there's a lot of problems with surveys, right, there's self-selection and various biases, and then, on top of that, there's not much rich context there.
And what we saw from call centers and various recordings every company has thousands of these conversations going on with their customers, with their patients, every day and they record it Like everything's recorded, right.
I'm sure you've heard the hey, this is going to be recorded for quality assurance and that kind of thing and a large percentage of companies. Just it just goes off into the archives so they don't actually do a whole lot with it, right, but it's an incredible resource. So actually our CEO was working, our CEO Amy Brown, she was running a call center. She was a CEO running a call center of I think it was a health insurance company at the time, and she of course saw this firsthand, day in and day out, that you get a lot of problems. But your customers are telling you what they are. Their customers are telling you what they need. They're telling you where they're stuck, where the problems exist. If you just listen if we would just listen to that, it would be you can make major changes. And so that's kind of where we started with that insight of the problem and the answers there, if you just listen to it. So that's kind of a little bit about the origin story there.
0:04:39 - Mehmet
Yeah, great Now. You mentioned at the beginning and of course, because now this has became more logical you said you use a lot of AI and the reason is you have a lot of data sets. But first let's understand a little bit about the domain specific AI, so like what domain specific AI is and how it's different from general other AI models.
0:05:04 - Michael
Yeah, well, that's an interesting question and, just like AI in general, this is evolving. I'm sure you've observed that AI has evolved very rapidly, particularly this year. I've heard the comment that, well, ai just started working six months ago, like when chat GPT-3 came on. It was like now we have AI that works. But domain specific AI really starts from the understanding that right now, as a company, if you're trying to build a company around AI, or AI is a big part of your moat, let's say, well, you have to realize that AI is kind of a commodity. Whatever the algorithms, the code that I have access to, everybody has access.
In general, there's just so much really really good open source that it's essentially just a commodity. And so we look at that and we say, well, if we're going to compete on that, what does that mean? What does that look like? And so there's really two different areas that we can focus on in the domain space. One is your training data. So if you have a unique set of data that you can train these models on, well, that gives your models this unique ability, unique sort of insight into what you're analyzing, and so that's a really big for us, that's a big part of our mode, and that's really what that's. A big part of what the domain AI looks like is your training it on very specific problems, and so that's really where we focus in.
0:06:47 - Mehmet
Yeah, now we talked about the call center, part of it, an application of the call center, but is there like and you talked about CX, which is basically, in this case it's the patient experience, right? So can you highlight some real world examples on how this could facilitate, maybe, the communication? Is it something that will make the diagnosis process easier? Like, what are exactly the use cases? I'm interested. It's very. I was on the website, actually on your website, and I saw like really some cool stuff over there, but I want to hear that directly from you, mikey.
0:07:28 - Michael
Yeah, yeah, definitely so. Well, so we right now we focus in three, the three big verticals. So we focus in on pharma, payers and providers, and even within healthcare, we narrow this domain specific AI even more. So we might look at payers or providers and say, well, we need to build a model to sort of solve this problem, and so we even sort of stratify it at an even finer level, generally speaking. But I'll give you a really good example of how we utilize AI and keep in mind that for us, the volume of data, like the scale of the data, is huge. We process terabytes every week of data. So we're talking about hundreds of thousands of audio files, potentially chat files, things like that. So the scale is pretty big, but one really. Here's a really unique model that we've developed that's really useful and really interesting. If you're familiar with an Eddy in a river, you've ever spent much time on rivers or anything like that.
0:08:37 - Mehmet
No, not really.
0:08:40 - Michael
Well, so it's kind of a slang term, I guess you could say. But when a river is flowing and there's a large boulder in the river or a large log in the river and the flow of the water is disrupt, so instead of flowing downriver, that water begins to sort of swirl or pool and it's no longer able to move downriver as it should, well, that's called an Eddy. So we've developed this concept of an Eddy effect, and really what it is is it's about friction. It's about you rein into a problem. You're trying to get, let's say, a procedure approved or paid for, or you're trying to figure out what's the process for a billing, payment or something like one of those lines, and so that's where this, what we call an Eddy effect, comes into play. So we have a model we've developed for the past couple of years that'll tell us hey, this conversation includes an Eddy, right, and so we can know that, hey, we've got friction. So we have clients that measure their Eddy effect rate across time, right, and so they're looking at this as well. In a way, they're looking at it as a replacement of NPS within this healthcare space, and that model itself is actually pretty interesting because it's actually pretty well, generalized across all the verticals. So at this point it understands how people talk about their problems, right, how they talk about hey, I'm stuck here, I can't find an answer those kinds of things. And so that's sort of a really foundational model for us, some foundational AI, because we can determine, like, hey, 20% of your interactions with patients have significant friction in them, for example, right, just as our start in point. And then for us, we build on top of that and again, we're very like we use AI in a really sort of really specific way where we're trying to solve really specific problems, and so we might then say, well, hey, within these 20% of interactions we have, eddie, here are the things we're talking about, right, and so you can then narrow it down one step further and say, okay, what processes do we have that really generate a lot of friction and a lot of problems with patients and clients? So that's really foundational for us as far as how we approach this problem.
Another one more example for you of like really specific model that we've built is what's known as safety events or adverse events. So in pharma, of course, indicating an adverse events are pretty big deal, right, where it needs to be captured and recorded, and so we have a model that can identify that hey, we've got a patient that's talking about an adverse event in this conversation. And then, of course, we actually have a follow-up model that indicates did your agent, did your customer representative, did they acknowledge that? Like, did they say, oh, okay, well, I better capture this information here.
So we're really tackling not just vertical specific AI, but we're also like problem specific AI, that we're really zero and not. And one of the reasons we do that is just like AI fundamentally, is probabilistic, right, like it's always the best guess, and for us that's not. That doesn't quite get it done in the healthcare space, like you have to have the right answer. And so we take something that's sort of fundamentally probabilistic and we try to make it as deterministic as we can. Right, like it has to give us the right answer, because that's what our clients demand, of course, but also just the space for it. We have to minimize any of that wiggle real quick.
0:12:33 - Mehmet
Yeah, and of course, like, because you in healthcare, it's like something that touched people's lives, so it has to be deterministic, of course. Now you mentioned something about you know the data sets and, of course, like this is something we try even to explain it to, to and to see us. About the AI and, to your point, like, ai is not chat GPT, that came out and the end of last year, so it's all about data and you know, storing the data and cleaning the data. So what I'm interested to draw from you, michael, is like data set creation, right. So for you, I know, like one one part of it is the conversations that happens, you know, during maybe calls, right. So Now, how for healthcare, specific AI models, this creation is important and how you can maintain and ensure that it stays relevant and useful.
0:13:33 - Michael
Yeah, that's a great question and of course I'm sure you've seen there's lots of interesting experiments done with chat, tpp, where you get some. You can really sort of get weird answers out of it, right, like very inconsistent and things like that, you know, and so that's a hard problem. But we've made a pretty big investment in actually developing and generating our data set, and so for us, it's not just the conversation, that's a part of the data set, it's also the labels, right. So we label. We actually have a team of healthcare professionals that we've hired their own staff and what they do is they're really focused on, in fact, labeling various interactions for us, and so through this exercise we've really generated this big set of data that we can train our models on, and it's not just labeling.
So, yeah, we hire people who know the space, they understand the context. But then we're very rigorous about calibrating. We have very regular sessions where we're calibrating on. You know, what is an eddy, what does an eddy sound like? And we're looking at examples and we're adjusting and we're tweaking, and so for us, that labeled data set is like really is really critical and a part of what we consider to be like a really unique asset really for us, you know.
0:15:02 - Mehmet
Yeah, so what are the challenges in this process, michael? I know think that comes top of mind is maybe privacy right, privacy of the data. So what other challenges you have when you do this?
0:15:16 - Michael
Oh yeah, I mean, privacy is a big deal. Privacy, data security those are all really critical items For us. It's, you know we're a, let's say, a younger company. You know we started in 2018, but that was also an advantage because just from day one, we built everything encrypted all the time. You know we use nothing but modern technology, and so I know you know there's other, let's say, competitors in the space, especially in the NLP space, who maybe been around a little bit longer. It's a little bit, you know, a little more difficult problem for them.
But you know we have some pretty strict rules about everything being encrypted. We have different teams actually that have been certified to listen to specific data. We've also put a lot of effort into actually redacting PHI, like you know, personal health information, and so that's actually a part of our process. We have a pretty extensive like data processing process and really one of the first steps is just to redact anything we don't need, you know. So that's actually just built into our process is just, you know, just eliminating anything that might sort of cause a problem or has a potential to cause a problem, and that's not easy, especially when you're talking about a conversation. Right, you're talking about very unstructured data. That in and of itself is, you know, is a challenging problem, but you know we also put a lot of development work into the reaction as well.
0:16:43 - Mehmet
Yeah, now, one thing that you know also, I noticed, you know, when I was exploring and preparing. So, of course, like you know, from industry perspective, you explained that. So it's like you know, pharma comes into the picture in addition to, you know, the hospitals and health providers. But from use case and role perspective, you know, I've seen, you know, in addition to patient experience, that's going so I've seen, like marketing and operation, so how that works exactly.
0:17:18 - Michael
Yeah, well, that's a good question. So one of the things we look at the conversation as this like multi-purpose, really rich data set right, and so out of a single conversation, the things that we can extract out of that begin with, like the agent quality. So that's sort of that's just like the baseline we can assess how well did the agent do? And that's kind of where a lot of companies are today as far as the maturity, their maturity is. They wanna assess how well did our agent do. But there's a lot more there than just the quality right. There's the customers. There's a lot to be going about your brand, for example, like how do they think about your brand, how do they feel about your brand, you know. So that's something that marketers are very interested in.
If we're hearing brand detractors or something along those lines, if you're a marketer you wanna hear that straight from your customer and you don't have to go do a survey if your customer is already telling you and of course we can extract that information as well. And then, from an operational standpoint, we hear about process problems. In fact, process problems are the majority. So we just did a study. Actually, we presented, we had our first conference a couple of weeks still called Voices, and so we presented a study at that conference and I don't remember the exact number, but it was a pretty big majority of the problems of the eddies that are indicated are related to process problems. So if you're in operations you can hear straight from the customer the process problems that exist and that they're really running into, causing a lot of friction.
0:19:04 - Mehmet
Yeah, so, michael, few moments ago you mentioned about when you do the training. You use labeling which is done by people who are experts, who they can put there, and labeling for people who are not familiar. It's kind of classification. If you want or input a tag like this, is that this is this, but what else? Ai trainings when I hear training, so this is the ultimate goal sometimes is to enhance the overall results, right, so does it go into a way where you want it to be kind of a and excuse me, because I'm not, like an AI, fully expert in this, but I know like there's a way where you go with the supervised learning model and then there's unsupervised learning model. So is this something that you do, so the algorithm or the AI can, by itself, goes and learn new things, or you need to keep feeding it with data and rely on the labeling for getting it back? Yeah, well?
0:20:11 - Michael
so I think the answer to that is yes. Well, at least from our standpoint, we look at every problem and say what's the best way to approach this? So we actually use supervised models, like you suggested, where you have a label, right, so you have an input, and you have that sort of output label that allows the machine to learn from right. So you can, and sometimes a lot. What we'll do is we might clip a little part of a conversation, let's say a 10 second clip, and we say, hey, in fact, this is one of the things we're working on right now. This is frustration, this is what frustration sounds like, this is the words, this is the tone, those kind of things, and so that would be a supervised approach, a supervised model. We also have algorithms that are unsupervised, where we're doing things like various cluster analysis, looking for different topics that might exist, might show up, right, we're looking for those latent patterns, but we're letting the machine find it right. So that would be an unsupervised type of approach. And of course, we've actually had.
We released our first language model two years ago, so our first language model was actually summarizing calls, and that's a self-supervised model, right? So you develop a language model by. Well, it's self-supervised, but really what it's doing, it sort of drops out a word, it predicts it, it says, hey, did I get it right? So that's the self-supervised approach. And even so we take all like we use all three of those approaches, but then we're always retraining whether it's like a reinforcement learning type of approach as we develop additional data.
The more the model can see and understand what the label is when it sees or hears this specific situation right, the smarter it gets, the better it gets, the more generalized it gets right, and so that's a process that we work on just really regularly, like our eddy effect model I think we just released version three of that and so for us it's a continuous improvement sort of process. It's not automated yet. We've considered that but as you've seen with chat GPT, it's a double-edged sword if you automate human feedback into the loop. So, right as of right now it's. You know, my ML engineers you know, and they do a little bit of analysis on the feedback data before we just say, hey, this is the right answer, go ahead and learn from it.
0:22:53 - Mehmet
Yeah, yeah. So, michael, you know I tried to follow a sequence to reach to this question Now as CTOs, you know, and even as consultants, because I was a consultant myself. So when we talk about a new technology or you know, when we try actually to solve a problem with a technology that we develop or we acquire or whatever. So we talked about metrics, right, so we talked about, you know how the business is going to be getting benefits. So, from your point of view, when your customers, or any customer I mean in this space adopt this technology, Like what are, I would say, in the short-term result that they start to see, and on the long run, what are also the benefits they see deploying, you know, this technology?
0:23:50 - Michael
Oh yeah, that's a good question. So usually our clients. So there's the maturity curve Because, again, this is not a space that's real common. You know a lot of enterprise companies have. You know, they have talent and they have experience with structured data. Maybe they have data warehouses, they have BI systems and you know, we're generating a lot of intelligence kind of similar to BI, but it's from this unstructured data, it's from these conversations, and so it's very new, usually to our clients.
A lot of them start with just hey, I want to assess the quality of my agents, right, I want to score the agents, I want to and I want to coach them. Like this is that's a big part of it is the action that comes out of the intelligence, that comes out of the AI and the structured data we're generating, and so one of the very first use cases you see is is that an improvement in the quality scores? You know so, a lot of times, agents are assessed on several different skill sets and you know, just, first of all, just by measuring the skill set, you start to see some improvement, right? And then one of the things we built in and this is actually part of our philosophy is, if we're going to build AI, we're going to build intelligence. We don't want to do it just because it's neat, you know, even though that's fun for some of us nerds, like we just like technology, but we have to be something we can act on. And so one of those things we've built in is this agent coaching capability. And so what you see is you begin to see those quality scores really improve as your agents are being measured and they're being coached and they're seeing specific examples of, hey, this would be a better way to handle that, or this is the answer. Or, you know, you can maybe find gaps in your training and things like that. And that's usually what you really see really early on, after you've implemented our software and begun to sort of utilize the program. And then, as you grow in your sophistication, you start tracking things like Eddie Effect. You start tracking it like you might track an NPS score and you want to follow that trend and begin to understand, okay, well, where are my process problems? And you begin to work on your process problems too, and you can track your Eddie score, your Eddie Effect score, and as really like, hey, let me see what the trend is and let's see how this is changing.
You know, we've had clients actually, too, where maybe they've had a new product launch and they want to know, like, what are people talking about? Like they just want to know what they're talking about, what are the topics, what's coming up? And so we see a lot of that coming up too, and to the point where we've had clients printing reports out of our system, taking it to the CEO once a week, taking it to board meetings, just because they want to know, like, what are your clients, what are they talking about, what are your patients talking about, what are the problems? And so it can be really powerful from that standpoint. And actually, I don't know if you saw, on our website we have something that's kind of unique. We call this the montage builder. Did you see that at all?
0:26:58 - Mehmet
Yes, I saw that.
0:26:59 - Michael
Okay, yeah, so I know this is about AI, but this is a really interesting thing for because if you've been in the data space, you've probably been in a situation where you see something that's like you get an analysis and you see this data that's really compelling right, and you want to share it with somebody else because you want to act on it right, like you want to say, hey, here's a problem, let's go improve it, but you can't get any traction, right. So I don't know if you've ever experienced that or seen something like that.
0:27:27 - Mehmet
It's amazing actually. I you know when, by the way, I have to say something like you designed actually the website in a way that is hooking Although, like I'm not, I don't work in healthcare, I work with them a lot but I mean like it's something that really touches people's life and actually the experience itself and it's all about you do so. The experience, because you know the frustration when you try even to book, maybe, an appointment with a doctor and you know when they let you wait on the line, or maybe you are checking, you did some tests and you want to see the results and you know, because something we do from time to time, all of us and when you see this, how you can enhance the whole process. It's really fascinating.
Now you mentioned a couple of things regarding the existing infrastructure, right, and the existing systems. So unstructured data oh my God, like one of my favorite topics. Like unstructured data, we'll not discuss the technical details, but I mean like how easy, or do you see it as a challenge, to integrate your solution, or maybe any AI solution, with existing infrastructure and existing other software? Because you mentioned data lakes. You mentioned maybe they are using some data analysis tool. So how is this, I would say, exercise to integrate something similar to your solution or AI in general from existing setups.
0:28:57 - Michael
Yeah, that's a great question and well, so let's just like just start with just the AI in general. I think we've been using this term lately. I don't know if this is offensive, but it just kind of describes some of the conversations and we've kind of referred to a lot of people looking to buy software as this kind of AI drunk right Like they're just like.
AI, I gotta have AI, right, like in the question, of course, like well, okay, well, what are you gonna do with it? What's the problem you're trying to address or how's it gonna help you? And that's we try to really focus on that. And I think right now it's really challenging, right, because there's. So it's like you gotta work your way through the hype and you gotta figure out, okay, where does it fit? This isn't a solar board. It's not like a magic wand kind of situation. It's powerful, but where does it fit? How can you use it?
And so, just from that point, we do a lot of education, and I'm sure there's other AI companies running into the same thing, because there's just so much hype around it. So that's a challenge. And then for us, the additional challenge on top of that is just the unstructured data. We've gotta get a hold of call recordings and of systems where they're, like they've been maybe storing them for decades, but nobody really has the knowledge of where it even exists, right. And so we've a pretty significant professional services where we help clients figure it out. And we help them figure out, like, where is this data, how do I integrate it? But then also, of course, how do I climb that learning curve of I've made this big investment. How do I get the benefit out of it? But yeah, it's, it's, it is. I mean. The truth is it's pretty challenging to figure it out. Everybody wants it.
0:30:41 - Mehmet
They just they're not sure what it is, you know exactly, by the way, like I had, like another AI company, they, they, they were facing the same challenge. When they talk to the customer and they say, okay, like we have this AI and the customer's first question okay, where is your chat GPT interface? Because now you know, everyone thinks like, when we talk about AI, okay, where is the chat interface? And you know it's not about the chat interface. But I think customers, slowly, slowly I mean especially enterprise customers they start to get, yeah, like it's not only about the chat.
Yeah, it's good, it's a good functionality to be able to extract data from the large language model, but I mean it's it's not like the whole thing, this is like just the interface. This is the top of the iceberg, I would say, and there's a lot of work that happens, you know, underneath. That needs to be taken care of. And yeah, good luck with extracting the data from from the Amstructure data platforms over there, like it's something. Really it's a hard exercise but it's not impossible. I would say so now you are in this space, michael, and you know what. What are you know the? I would say it's maybe like a traditional question, but what are you know the trends you are seeing in that space, in the health care and AI machine learning space, like what? What other use cases you're seeing happening?
0:32:12 - Michael
Yeah, well, so so a couple other use cases and these actually would be really good use cases for large language models is just being able to answer questions. You know, and I think this is still really, you know, not not very mature at all, but you know so many patients and and and customers like they just have a question they need an answer to and oftentimes that question is it's a simple question but the answer is kind of complex or it requires a pretty detailed set of knowledge around your policies or protocols and not even necessarily like like health knowledge or you know, it's just like what are the rules you know, and so I think that's that's an area I would see, like you know, just ancillary to my space that I'm focused on. That would be just right for AI to really be beneficial. We are hearing a lot of talk from from clients that they think they think AI can replace their call centers, they can replace all their agents, replace all their call centers. I'm not. I wouldn't be in that camp, I think.
I think people still want to talk to people, you know, especially in healthcare, especially when you're talking about your health you're talking about well, in a lot of cases you're even talking about life or death. You want to talk about other human being, and so I don't. I don't think that's that's the situation we're in, but there's a lot of, there's a lot of talk about it, of just replacing humans and how can you replace people. That's not really. We come down. We really come down on how can we augment it, how can we experience better, how can we augment humans, how can we help them do their job better? But but there's a lot, there's a lot of talk of replacing people right now.
0:34:01 - Mehmet
Yeah, but I am. You know we discuss also this on the show and especially in the healthcare. Like I asked the question, are we going to see even, you know, ai replacing physicians? No, of course not, but you know it will empower, you know, whoever is using this technology and I'm fan of you know, being AI powered. I would say so. I'm just thinking now of a use case. So if someone is calling and you know there's these results that are written by an MD, you know describing, you know what the results are and usually they are very scientific. So maybe, using the AI, the agent can use the AI to translate this into, as we say, the layman terms to the patient, like, hey, you know that means blah, blah, blah. You know in a very more simple way. So maybe it's, maybe it's a use case. I see it. Yeah, replacing, yeah, unfortunately, like some of the jobs would be replaced, like there's no you know, escape from that.
But it's about elevating. You know the scales and getting you know to to to the next level. Now, as we are coming almost to the end, michael, like I want to to ask you something regarding being a CTO in this space, like how it's different from being a CTO in any other space and what advice you give for someone you know he or she might be interested in going this direction that you went, like any, any hints or tips you would give?
0:35:32 - Michael
Yeah, that's well. So we, for some reason, we decided to find one of the hardest spaces to tackle. So we're not just in healthcare, we're also tackling the we sound to the biggest enterprises in healthcare. I was actually just at a summit last week with other founders and I think almost everyone said, well, we're avoiding enterprise, we're in small business or meet, you know, in the medium company space, and we've got. Well, that's not us, we're like what's the hardest possible we could go after. I think we might have found it.
You know, and one of the things that's really been beneficial for us is to go in with and be very security minded. So we put a lot of effort into data security and privacy and you know, as we're working through, you know, the security questions and the security assessments, each one of our clients, we often get the feedback like hey, that was the easiest you know, assessment we've done and it's because we put a lot of effort and thought into it. You know, I've got my security guy, ken. Ken Getch is just, he's all over this, you know, he's just, he just loves his stuff, he's just, he's just awesome at it and so.
But that's really been a bit of an advantage and so I think if you really want to get into this space, it's not necessarily the fun part of it. You know. I know for me like I'm all about, like I want to talk to my email engineers, I want to talk to my app engineers. You know that's the fun, that's the fun stuff. But get your data security right. You know it's just. You know, just from the beginning, just just make it so that it's not an issue and that'll definitely smooth the way. You know it may not be the fun stuff, but what make it happen to be in better?
0:37:18 - Mehmet
shape. Yeah, cool, like I know. I think it's a very valid advice of it and if you're passionate about it, go for it. You know, whether it's this space or any other space is what I tell. Even you know entrepreneurs and founders that they come to me sometimes. So, michael, is there anything that I should have discussed, asked and I missed?
0:37:43 - Michael
You know. There's only one other thing I wanted to add is, just, we talked about AI and we talked a lot about data. One of the things we're actually really careful about, especially in customer experience space, is we're generating data, we're generating output from AI, but we always try to lead our customers back to the literal voice of the customer. We want them to hear the patient, we want them to hear what the patient of the customer has to say. We want them to hear that.
You know that might be frustration or pain in the voice. So, even though our AI is telling you X, there's just no replacement for actually hearing it for yourself. And so, at the end of the day, for us it's all about, like, we want to be really careful about not dehumanizing by using AI. Right, we want to make sure we're still focused on the human beings that are involved in this process, and so I guess that's something I want to share, but also just I would encourage others who really want to get in the space, especially from a healthcare perspective, is just, you know, don't lose sight of that, that sort of human aspect of it.
0:38:49 - Mehmet
Yeah, 100%, michael, and thank you for bringing this in. And because we discussed, you know, when we talked, I had some episodes just dedicated for customer experience and you know also which makes sometimes with the digital transformation things, and we said, like you should not usually rush to implement any technology, whether it's AI or any other technology, because, especially in cold centers, we heard like horror stories about, you know, when they put bots and you know customers were frustrated because they were not able to talk to a human and you know they were all what they were getting is okay, if you have this press one, if you have this press two, press three, and it's like it's a look. There's no escape from that and they had to get frustrated and yeah it's a very valid advice and thank you for bringing this, Michael.
So, Michael, when we can find more about you and about your company?
0:39:51 - Michael
Yeah, we so. Yeah, you can check us out at Authenticscom. We've got a lot of stuff out there, a lot of case studies. It's pretty interesting. Of course, I'm on LinkedIn, you know, so you can find me there. I don't really I don't tweet a lot. Amy Brown, our CEO, tells me that I'm too provocative, so I try to be a little bit careful about that.
0:40:18 - Mehmet
Yeah, that's completely fine. Cool, I would make sure that all you know the links. The company website is in the show notes, of course, and if someone wants to connect with you and if anyone has even question to Michael, you know you can send to me. I will pass it to Michael. Michael, very much for you know the time and for being on the show. It was really informative.
We saw today a different, I would say, or let's say we looked at a different angle from the customer experience slash, ar, which is mainly for the domain that you serve, which is, you know, health care, insurance companies and so on, and even like use cases, which are guys. I advise you to go and check because this is something you know I didn't maybe discuss it before enough. You know about the patient experience, which is very important and it touched all of us. So cool stuff. Again, thank you, michael, for being here today and for the audience, thank you for tuning in and thank you for sending your feedbacks, your questions. I really appreciate that. If you're interested also to be a guest on the show, don't hesitate, reach out to me. We can arrange for that as well. If you have a cool idea, you have a cool thing you want to discuss. And yeah, thank you very much and see you soon. Bye-bye.
Transcribed by https://podium.page