#296 Mastering the Art of Managing AI with Robert Plotkin
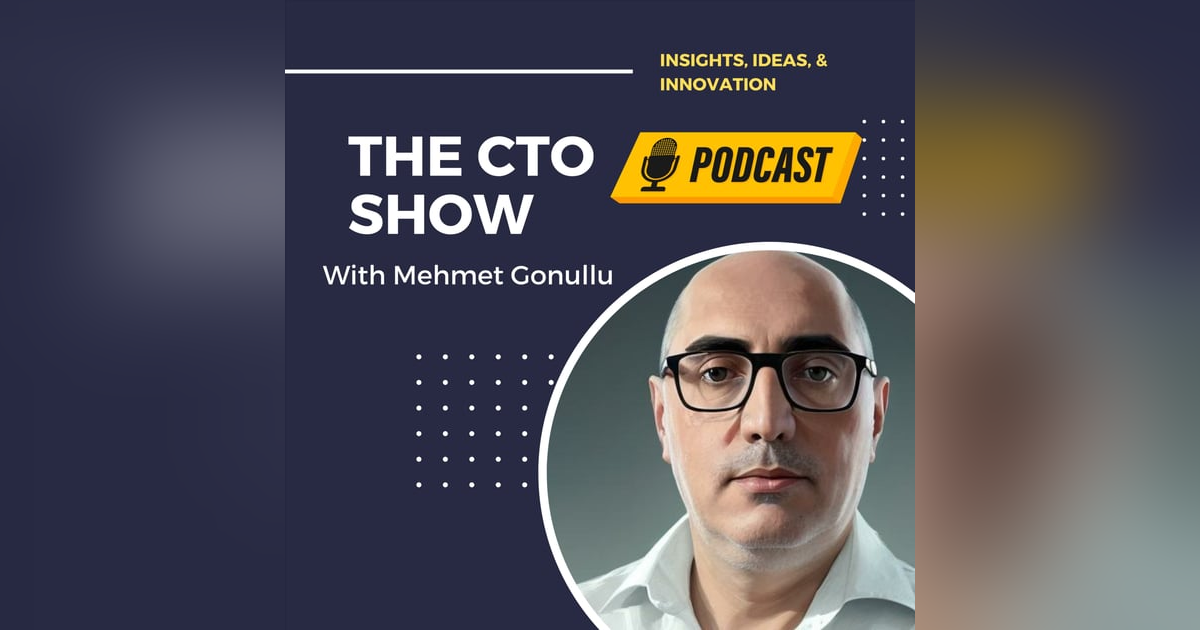
Unlock the transformative power of AI with our esteemed guest Robert Plotkin, the sharp-witted patent attorney who's once again gracing our studio with his insight on the latest AI upheavals. This episode sails through the emergence of chat GPT and its role in the democratization of AI, making powerful tools like natural language processing accessible to the masses. We dissect the daily advancements in AI, showcasing how these technologies arm professionals from myriad fields with capabilities that were once the stuff of sci-fi dreams.
As the conversation progresses, we navigate the intricate dance between AI bots' burgeoning autonomy and the crucial need for human oversight. Robert and I tackle the evolution of AI interaction, addressing the challenge of "AI drift" and the vital role of human-guided management—a concept as intrinsic to AI as it is to traditional business practices. We also confront the misconceptions about AI, separating hype from reality in an effort to understand the actual scope of AI's capabilities and the measured approach needed to harness its full power responsibly.
Wrapping up, we venture into the practicalities of AI implementation, weighing the perils of both overestimating and underestimating its potential. By drawing parallels to the utilization of junior lawyers in legal practices, we advocate for valuing practical utility over the quest for human-like reasoning. We also examine the 'jagged frontier' of AI—the unevenly trained athlete of technology—and emphasize the importance of error checking and refinement to exploit the speed and efficiency of AI, all while keeping inaccuracies at bay. Join us for this illuminating session that promises to broaden your perspectives on AI as a valuable ally in the modern professional landscape.
More about Robert:
Robert leads innovators, creatives, and professionals in leveraging AI to boost their skills, effectiveness, and productivity to the next level. Robert brings an optimism and excitement to his work that are the perfect antidote to the fear of being replaced by AI.
https://robertplotkin.com
00:45 Introduction and Guest Reintroduction
03:37 Discussing the Rapid Advancements in AI
08:44 The Role of Humans in the AI Era
14:50 The Future of AI: Opportunities and Threats
22:21 Understanding the Capabilities of AI
30:27 Understanding the Functionality of Large Language Models
32:16 The Role of Reasoning in AI
33:39 The Imperfections of Human and Machine Outputs
34:47 Exploring the Transformer Paper Behind GPT
36:39 The Concept of Reasoning in AI
38:34 Addressing the Accuracy of LLM Output
39:02 The Evolution of AI Tools and Their Applications
41:18 The Challenges of Trusting AI Outputs
49:04 Learning from the History of Technological Advancements
56:07 Closing Thoughts and Contact Information
0:00:01 - Mehmet Hello and welcome back to a new episode of the CTO show with Mehmet. Today I'm very pleased to welcome a guest that was with me actually in episode number 114. That was last year, and you know this is the third time I'm bringing previous guests because, you know, at that time the time wasn't enough for us to discuss and especially because Robert is expert in the field of AI and you know, at that time we discussed a lot and you know, chat GPT wasn't new. Robert, thank you very much for being with me again today and thank you for your time, just for people who, you know, maybe they didn't see the first episode. If you just, you know, reintroduce yourself what you are up to these days. 0:00:49 - Robert Yeah, absolutely. First of all, Mehmet, thanks so much for having me back and congratulations on the great success of the podcast. I follow it regularly. You're really doing a great service to the public and the community by hosting all of these episodes. 0:01:06 - Mehmet Thank you. 0:01:07 - Robert You're welcome. I am a patent attorney. I specialize in obtaining and forcing, maintaining patents for software and AI. I've been doing that for over 25 years and it's a super exciting time to be in the field and seeing everything come to fruition. I am a co-founder of the law firm of Blue Shift IP software patent experts. In addition, I love talking about how to use AI, to leverage AI in your own work, whether it be as a professional like a lawyer or a doctor or a marketer or writer or podcaster, and how to use AI to become more productive, efficient, and how to supplement and complement and boost your creativity. So I'm glad to talk about any of those topics today again sure to be in the AI world, where there's so much available to really use it is and you know, you know before we start and before you joined as well. 0:02:13 - Mehmet So during the day, I was reviewing what we have discussed and this is, like you know, it's like just a couple of months back, it's not like two or three years back and a lot of things have changed in less than one year. So you know from your experience, you know what you've seen and, because you know, I remember, like both of us, how we were very excited about the AI. So first, before we dig into you know, the use cases you just mentioned, like, have you seen, like Robert, anything like this before about this fast shift and this adoption of AI? I just want to see how you, I would say, evaluate. You know what happened with AI last year, during 2023. 0:03:07 - Robert Yeah, I have never seen any pace of innovation like this that we're experiencing now. You know, I've been using computers since the early 80s and you can think about some previous revolutions we've experienced. There was the personal computer absolutely incredible, you know, brought computing power to the average person's home at a low cost absolutely incredible. Then there was multimedia. There was the internet, you know, starting. Although the internet was around since the 60s or the 70s, we usually talk about the introduction of the web in the mid 90s as the start of the commercial and public internet revolution absolutely incredible. Smartphones and mobile devices, you know, around late 2008, 2009. All of those things are absolutely incredible. And yet I would say that what we're experiencing with AI is is much bigger and much faster in its ability to reach more people more quickly and impact people's work, and ability to access and understand information and to get work done. I mean, although the tools underlying something like chat GPT were around for a long time about a year ago, end of November 2022 which I call the dividing line between before chat GPT, bc chat, chat GPT and after chat GPT, because it was such a stark line that put into the hands of anyone with an internet connection for free, the ability not just to access information, which you could say was around since search engines became available but the ability to summarize, synthesize information, explain information, but also and this is why we call it generative AI to create new information, to start to perform tasks based on instructions, and all using natural language that anyone can use. So you could obviously provide a natural language instruction to chat GPT. Find me a recipe or write me a poem. I can know. Those are two different things. One of them is to extract existing information. Another one is to synthesize new information. We can argue about the dividing line between those two, and a lot of people do, but then to so provide that natural language input and to get clear, easy to understand natural language output, I mean that's what's been so absolutely revolutionary is to take the, the types of AI tools that were previously available only to technical experts who understood how to program using either programming languages or otherwise very highly technical ways, brought that into the hands of the average person in an easy to use way, for free, from any device. I mean that's just absolutely incredible. Now we can talk next about what's happened since then. As you said, even if that's all that happened and nothing improved since day one of chat GPT, I'd say we'd be dealing with the ramifications of that for a very, very long time and yet the advancements that have happened in that last year are just just absolutely incredible, and they're coming about on a nearly daily basis. That's the next part. When you ask me have I seen anything like this before? I mean, the personal computer revolution happened over the course of a decade or two. Even the internet, because fast as it seemed to happen with the web, you know, the web grew and improved over many, many years. These improvements were seen with AI, are rolling out on a nearly daily basis and sometimes they're very significant improvements, often to tools, again, that are available at low or no cost to anyone and that are easy to use by anybody with little or no technical skill, which is part of what's being called the democratization of AI, meaning bringing the power of all this available to a much, much larger range of people than could ever use it before. I'll stop there, because you know there's a lot of directions we could go from there, yeah, absolutely. 0:07:38 - Mehmet Now, what I want to start with you know and this is something we talked about last, last time we spoke, and actually you know it was something that kept coming every time I was speaking to an AI expert or enthusiast, and and even, you know, sometimes myself, when I was sharing some, some articles here and there. Now I know you, you have you have wrote an article called turning the tables and you talk about professionals evolving into supervisory roles over AI and this is, you know, like when I read the article, it, it attracted me. You know even the, the title. You know it's very hooking actually now, but I want you from, from your, because you, you wrote this and, of course, you, you did some studies on that. I want to to describe to us, robert, you know, what qualities and skills are crucial for people like us, professionals, to successfully manage and work alongside AI. Because you know, I remember very well when you know we said, like the people who would be able to master AI will be able to thrive, and so on. But you know, now you started to talk about managing and working alongside the AI system. So I want to a little bit describe, you know the qualities and skills here that we need to have yeah, absolutely. 0:09:06 - Robert I mean. One thing that's always a topic of any conversation about AI is the fear of being replaced by AI. It comes up every time I talk to someone. People are afraid they may not. You know, if they're a business person or a programmer, they may not use the word fear because it might be an embarrassing thing to admit so. They phrase it in other ways, but underlying a lot of the discussion is fear. You know you can look to science fiction. We have so much of it. A terminator, it often comes up the matrix. So much science fiction and mythology plays on and and and attends to this fear we have that we are going to create technology that's going to become so powerful that humans will either just become obsolete or will actually be replaced in some more nefarious way by technology, and so one of the motivations for this article was to point out how Humans will always be needed. There will always be an important, valuable place for humans, but it's also true that AI is rapidly automating a lot of skills that humans have been valued for in the past, in the context of writing, for example. Something like JackGPT and other large language model based tools are quite good at being at least average writers, and they're getting better. They're getting really good at writing basic code For lawyers. They're becoming quite good at summarizing documents, finding errors in documents, even suggesting legal arguments. This is a pretty significant fraction of what lawyers often do. So the point I make in the article is that if you want to remain valuable actually become even more valuable as a human and not be replaced by AI you're gonna have to learn a new set of skills to supplement your own set of skills, and the way I framed this was to say, as a guideline for always thinking about what are those skills you'll need to learn. Think about yourself and your current job whatever that job is again writer, marketer, programmer, lawyer and ask what are the skills that your current boss or supervisor needs to have to supervise you effectively? It might be to give you clear guidance about the task that person needs you to perform. It might be to give you background materials, documents, studies, procedures that you should follow when performing the task. Ask what are those skills your boss should need to supervise you? Well, those are the skills you should be focusing on developing now so that, when AI becomes capable of doing the tasks that you are now performing, you will be able to supervise it as your effective replacement. I'm saying that metaphorically. Jackgpt doesn't just wake up in the morning and do tasks on its own. It needs to be given guidance and instructions in the form of prompts, and not all prompts are equally good at getting the job done. There's a lot of difference in the quality of the output you get based on the quality of the prompts you provide. So the article goes through a sequence of examples for different types of job roles about the kinds of skills you will benefit from to become an effective supervisor of the AI. That might otherwise or may actually replace you, but so that once you have those skills, by being able to supervise the AI, you'll both remain valuable but become even more effective. And the last part I'll make on that is that by retaining the old skills you've had, you'll become even more effective, because the AI is never gonna get the whole job done for you, as we know. Keep using JackGPT as an example. It's pretty good at a lot of things. It's quite bad at a lot of things. It makes mistakes, it makes errors, its output needs to be fixed, refined, massaged in various ways, supplemented. So if you can gain those new supervisory skills the ability to give instructions effectively to AI and also manually revise its outputs, manually create other outputs and know when to use the old skills and the new AI supervisory skills you will both be more effective and productive and be more about put yourself at the top of the value chain. 0:14:06 - Mehmet That's wonderful. Now, one thing, because when we started the discussion you talked about and this is a lot of my guests also brought it and even myself I was excited about it about the idea of having multiple AI bots, agents, working for you. Now, do you think, robert, that and this is the way I understood from you we're not talking anymore about replacing jobs, but it's becoming, as everyone becomes, kind of a, as you said, supervisor or manager? Now people might see this as opportunity and some people might see this as a threat. So, in your opinion, can we see where everyone has a title manager, because actually they are managing these bots? And remember, the bots that we're talking, the AI bots, actually are kind of a specialized team. So you might and actually I've spoke to maybe three or four founders who they focused all their efforts on building specialized bots in specific area, for example, an AI bot who does only finance and other one that does only legal, like another, and then they try to bring all these together and it's kind of everyone needs to become kind of a chief operating officer, kind of these skills. But again, from your perspective, do you see this as an opportunity or do you see this as a threat, because maybe someone will say, hey, but not everyone can become a leader or supervisor. So what's your take on this? 0:16:04 - Robert Yeah, I mean it is a threat and it is an opportunity. It's both. You know, every threat is an opportunity or you can choose to see every threat as an opportunity. So I'm not gonna say it's not a threat and that's why I say, if you want to avoid being replaced when AI becomes increasingly capable of automating specific skills that you previously performed yourself manually, then you need to learn the new skills for supervising. The bot issue is really interesting. I know a lot of people, as you said, are saying that that's the next stage is to develop more automated bots. You know, because something like chatGPT is. It automates a certain task but still requires a human to provide every single input and guide it at every step along the way. So the next level would be bots that, as you said, either are specific to a particular domain, like finance or law, or booking meetings or things like that, and then the next stage everyone's talking about is connecting all those bots up together to communicate and interact with each other autonomously. I am sure that that's going to be happening. It's a big open question how quickly that's going, particularly the latter part, the interaction of multiple autonomous agents that perform different tasks. You know how quickly that's gonna become feasible in a way that's really productive. I don't know. You know I don't have a crystal ball, but I suspect that that's going to require overcoming more technical hurdles and may take longer than some people think, because the human guidance for now is still super valuable for both keeping the AI on task and for keeping it from just generally going too far astray. You know, I'll use a specific example as an easy to understand metaphor, which is you've probably seen a lot of the tools out now that can generate video, right. So we've gone from things like dolly and mid-journey, which can create a single image from text. It's quite amazing. And then I talk about what's happened over the last year. I've seen people post images they created using a single prompt in mid-journey, you know, from version four to five, 5.16. And you can see that how much incredibly better the images have gotten over time from the same prompt, just from the image generator improving over the course of the year, which is an amazingly short amount of time. So, but then there's now tools that will go from just a single image that you give it. You give it an image of a dog and you say generate video and it'll generate a six seconds video of that dog running. You know, whatever it thinks was the logical motion to generate based on the initial image. The challenge that those image generators have in going much further than a few seconds is they don't know, so to speak, what direction to keep taking the video in, and they start veering off further and further from the initial video, which might be fine, but in most cases after 20 seconds you end up with something that's not what you as the human really wanted or needed out of the output, and so that general problem of drift is something that's an issue with all kinds of bots. Once we let them just run free for too long or too far, I mean, they might do things that are productive in some sense, but that might not be what you as the human wanted or needed out of it. So I suspect that that's why it's going to be valuable, or perhaps even necessary, to have humans in the loop, whether it be for a special purpose bot over time, or certainly for multiple autonomous agents, because they're just going to start going far afield of what we want or need or what's useful for us. And that I actually find this fascinating the problem of how to design processes and skills, habits, routines for human, computer or human AI interaction, particularly in that context of autonomous bots. What are the best ways for humans to, let's say, be monitoring a set of box and course correcting them over time as they go off course, giving them new input or feedback. It's another example of what I've been calling the supervising, but I don't think we know a lot yet about what the best practices are for that, and we're probably not going to be able to know too much until the systems get evolved and we can experiment with them. It's going to be a really fascinating time, though I think what's interesting is, you know, we can draw from what people have learned in business management theory, right and practice because it's very much analogous to working with large teams, small or large teams of people in a business. So if we're going to see interesting and strange ways in which knowledge from from diverse fields like computer science and management all come together to produce new, more effective outcomes is going to be a fascinating time. 0:21:38 - Mehmet I again, I agree with you, robert, and you know, like the discussions again, and you know, some of my guests and some of people, of course, outside, you know they think this will happen very, very fast. You know this. And some people they say, no, we still need some time. Some of my guests, they believe that you know, and you know like the problem is. You know, like this, this topic it's very debatable and again I relate to the next question, but what I wanted to say some people they say already you know what they talk about the artificial generative general. I, actually it's already here, right. Some people they say this because you know, to your point, like now, everything we can even think about it. Back in the days, we used to say Google it or Bing it or whatever search engine, and now go find an AI to do this and, honestly speaking, what I'm seeing is there is a fast pace in in the way things are moving. But and this is how I'm relating to the next question I think and you covered this in another article and I love that one when I read it about, you know, actually sometimes we see people they don't understand actually what is this whole AI is and to your point about, for example, when you mentioned about the video tools, that they can just do few things. But people have this misconception about AI, right, and you wrote the article called LLMs just don't understand so what right? So, and I love that because, again, even with founders who are building things related to AI, somehow the problem is they. You know, they told me always our problem is, when we go to people and they and we say I tool, their first thing that comes to their mind is the chat GPT thing. Right, and you know, and we forget about, like the chat GPT is actually built on something which called the transformer model, which is, you know, one of the LLMs large language models out there, and you know, like there is a lack of consciousness understanding. So I want you, robert, to to a little bit shed some light on this part, right about you know the LLMs capabilities, but still you know how we can, we can, you know, use them. And because in that article, like you were mentioning some terms like merely just you know, which are like people, sometimes they put too much hope. Do you think on on what this I currently it can do? So if you can a little bit, like you know, dissect that, that one. 0:24:30 - Robert Yeah, I mean you raise some great points, which is we have. You know, there are some people and they often are founders, understandably, because they see the incredible promise of AI. So there's some people who I think go too far in assuming that AI is going to be able to do too much too quickly. But you know, I think sometimes that's what's needed to drive people to put in all of the time and energy they need to put in to be found. So those people, I think they're going too far, kind of over promising what I can do. And then there's people on the other end and I see a lot of this amongst lawyers who think that because AI isn't perfect or can't do everything, they just shouldn't bother using it for any purpose at all. And those, those are two extremes which I think are counterproductive. And if you promise too much, I think you run the risk of contributing to a hype cycle in which the public relies on your promises and then inevitably becomes disappointed. And that's happened so many times in the history of technology, certainly not just in AI, but definitely in AI. I mean, maybe some of your listeners are familiar with the term AI winter. There's been a few of them over the past few decades, you know, starting in the 50s, when people there was a conference at Dartmouth in, I think, 1956, it was the place where the term AI was coined and there were some brilliant people there who thought that over the course of a summer they were going to be able to solve what we now call natural language processing. 0:25:56 - Mehmet you know, it was a little bit too ambitious. 0:25:58 - Robert They didn't understand how difficult it was going to be. But people in the field kept making these promises. Don't worry, general artificial intelligence is just around the corner and the public and investors and, you know, large companies were relying on these promises. And then they failed to come to come to fruition. And then what happened? You know we'd go through an AI winter, meaning people stopped investing in AI. People heard the term AI and said I don't believe that, that's not real, it's not going to work. I like the old fairy tale of the boy who cried wolf, where people just don't believe you when you keep making promises that you can't follow through on. So that's the first risk. But on the other side, I mentioned, you know, I'll point out, my my colleagues, many lawyers who look at something like chat GPT and say, oh, I asked it a question and the answer contains a hallucination. It contains a citation to a legal decision that is not real, that doesn't exist. Therefore, I will not use this tool for any purpose and I think that that is short sided and actually counterproductive. What do I mean by counterproductive? I mean that person stands to benefit significantly from chat GPT if they could come to an understanding of what its strengths and its weaknesses are, because then you can use a tool like that for for its strengths, what are? What are some of its strengths? These tools can often summarize large documents really well not perfectly, but really well. That's super helpful and I would I often point to the analogy in the real world. As a lawyer, for example, if you have a junior lawyer or a law student as an intern and you ask them to summarize a document, don't you know that they're not going to give you a perfect, 100% accurate summary? And if the answer to that is yes, I guess, well, why do you have them do that task? Why don't you just say I'm never going to use such a person to do a task for me? It's because you you do the cost benefit analysis first of all and you say having that person to that test might save me two hours of time. I know I'm going to need to spend 10 minutes maybe fact checking what they've done, but that overall process of me saving two hours and spending 10 minutes. Fact checking is to my benefit compared to me having to do all of the work. So think of of tools like chat GT in the same way. Look, try to. You need to learn about what their strengths and weaknesses are, and some of those strengths and weaknesses are not the same as the strengths and weaknesses of either prior tools like search engines or the strings excuse me strengths and weaknesses of human assistance. So it does take some time to learn, but once you can learn that those strengths and weaknesses, the speed and generally high quality but not perfect quality of LLMs is so significant that you can get real gains from them. Now let me turn to the arc about LLMs. Don't understand. The point of that was to address a very, very common criticism. 0:29:23 - Mehmet I see and this is not just for lawyers. 0:29:25 - Robert There are some people in the computer science and AI world who say an LLM does not engage in reasoning. If I ask it to address, to solve a problem and it gives me an answer where the output is accurate, how should I? If I want to go to the grocery store and carry more stuff than I can carry in one trip from the store to my car, what should I do? It gives a pretty good answer. Well, there are people who say but you know what? It's just parroting back text that it's seen before in the training data. It's not engaging in reasoning and therefore you can't rely on it. So there again, I would say well, having almost too much knowledge about how the LLM works can sabotage you. Because if, in many cases, an LLM can provide you with an answer that is accurate, why does it matter whether it created that answer by reasoning or created that answer through a process in which the LLM understood what it was doing? As long as the output is useful to you and as long as you have some procedure or set of tactics in your bag for verifying or checking the accuracy, why does it matter? Computers generally outside of AI don't understand what they're doing. Computers internally are really just a very large set of switches that turn on and off in response to the way we program them and the inputs we give them. They don't understand anything of what they're doing, but we've learned over the years how to program them in a way that, when we give them an input and get an output. The output is accurate or accurate enough that the speed gain and the automation gain is great enough that we can rely on the output. I think part of the reason why people are so focused on arguing that LLMs don't understand and can't engage in reasoning is some sort of unstated or unconscious anthropomorphism or some I would call it ego, almost some belief that we as humans have some unique hold on reasoning and we want to hold on to our own value at doing that. And then, when we see that LLMs can't reason, we can point to that fact as a way of protecting or defending our own turf in the world of reasoning and logic. But I would suggest let's try to let go of that ego and just get the benefits, the practical benefits we can, out of LLMs. It's true that their lack of ability to reason can lead them to produce incorrect results, so then let's just find ways to catch those results when they're incorrect and fix them. That's what businesses do all the time. I hate to say it, but businesses employ us as humans who are far from perfect. None of us produces perfect, accurate output all the time. You know what? I'll let you know the secret. We as humans often produce a work product that wasn't based on reasoning, is based on habit or hunches or intuition or bias or all of these things, and so a good business still employs all of us as humans, knowing that. And it puts procedures into place for checking our work, enabling us to check each other's work, check our work using computers, using spell checkers, grammar checkers, databases, search all kinds of things, so that the overall process is still as accurate as it can be in light of the imperfect human and machine components that make that process up. And once you see it that way, I think the fact that LLMs don't know what they're doing, aren't conscious, don't engage in reasoning all becomes largely irrelevant. 0:33:46 - Mehmet And I have an advice, which is you know, I did it myself at some stage, so I went to chat GPT. Now, okay, you can do this and you can understand more, so I wrote this. I can put it in the show notes also as well. Type this ask chat GPT, this. What is the transformer paper about? That was behind GPT, and you know it will tell you about this paper, which was actually developed by researchers at Google, funny enough who were in deep mind, and the title of you know the paper is attention is all you need, right? So so I advise everyone and, because you know what chat GPT will try to explain to you in a, in simple terms, right, so this is the, so it will explain to you how actually the LLM and you know GPT is good one, because this is what the world knows, and we know there's a lot of other models, but this is what you know. Every single one of us, I believe they, they, you know they, they they've seen, because of chat GPT, open AI, and you will get exactly what you are explaining, robert, like, about how this works, and actually you know what the researchers are trying to do, or try to actually they achieve, is to mimic the way we humans we act. Because when you, I remember when I was at school, you know, and I think everyone you know would remember when they used to give us this large, you know, piece of of literature, whatever it is, and they used to tell you, okay, go and write a summary about it. So, and you know, they used to highlight you need to use different words, you need to rephrase things, you need to. You know, and this is actually what GPT does. You know, it takes the input. That you know, we know it's, it's actually trained for this. Now, what they have done is that they added, you know, you know this, some people they don't like to call it reasoning, and I can understand, but you know, we need to also convey that the word reasoning here it's different from consciousness, right, robert? Like it's not the same as we say. You know, conscious we are the conscious ones because we give the prompt to chat GPT, but the reasoning here and sorry if I'm taking the, you know the, the, the lights from your Robert, because it's a very important one. So simple example, and about an AI case not related to chat GPT. So if you play any board game, let's say chess, so you do some calculations, whatever you want to call it. In your mind you start to calculate the steps. If I do this, you know the other guy will do that, and this is like the reasoning. You're trying to expect what the opponent will do and based on that, you, you move your piece and actually when you take this, you put it into a you know software or like program. Actually you are mimicking the reasoning of a human and actually the machine, because it's much, it has much faster capabilities. Actually it's only performing this task at that time. It does it very well and it does it very fast. And this is why you know everyone maybe remember when the IBM, you know, program one over the Kasparov, right? So it was the big thing at that time. So it's the same. It's the same thing with with chat, gpt. So when we say reasoning, you know, and I you know, this is why I also get too much excited about this article from you, robert, you know, and this misconception, reasoning is not consciousness, it's something else, right? So 100% on that one with you. Now you gave some, you know, you mentioned some advice on how we can, I would say, make, you know, make corrections. So in your opinion. You know how we can address the accuracy of the LLM output, so you suggest developing manual procedures, creating systems. So what steps really? Organization, because you are talking business, right. So what if I'm a business owner today? Or maybe I'm a chief AI officer? I'm responsible for the AI adoption at my organizations. What are the things that I can, I can do? 0:38:18 - Robert The first thing is really positive news is that there are companies out there addressing this problem with technology right now. I think in we're already seeing, I'll say, for example, in the law there are some legal specific products for things like doing legal research or drafting or revising contracts. You know you mentioned these domain specific bots. You know these are very domain specific AI tools that are combining language models with databases and rules and error checking algorithms essentially putting all these things together to address the problem of what is locally called hallucinations, or you could just call inaccuracies that result from LLMs. I think in the near term, that's a really good way to address the problem is to have domain specific applications that have been designed to have what we're calling error checking built in. So in the case I'll give a specific example go back to writing a legal brief a tool that uses both an LLM to do the drafting but that has access to a database of legal cases that it can use to either generate or check the citations to make sure that they are accurate and reflect real court decisions and are not hallucinations. Those two things can be married together in a smart way to produce a tool that gets the best of both worlds the best of the summarizing, generative aspect of a large language model and the best of the accuracy of an old fashioned database search or search engine. Those are some of them again already on the market and I suspect in the next year we're going to see a plurry of more of those domain specific tools, because that's the low hanging fruit is to apply the. You know that that type of approach in specific domains and the great news for businesses that are not, you know, software development businesses is they can then license an external tool and not have to worry about developing one on their own. Let me just say you know, there is an aspect of the inaccuracy what we call it, that of LLMs that is different in a way than what we've seen in previous generations of technology, which is that when you use a tool like chatGPT to produce an output, there's there's well, there's actually two things. One is there's no clear indication of what its sources are. So that can make it a lot more difficult to either know when you need to check accuracy or to engage in that checking of accuracy. If you use Google to do a search and it comes up with 50 websites, you might look at what those sites are and just use the source as a heuristic for what to check. So maybe there's certain newspapers you trust more than some no-name website and you can tell that immediately in your Google search results. A LLM just churns through text and produces it and doesn't tell you where it got any of this information from. So if there's, in a variety of ways, there's often no clear cues that the output of an LLM gives you as a human to trigger you to raise your radar and say how this is something I maybe shouldn't rely on too well. So that means for individuals and for businesses, you just have to adopt more of a general trust, but verify attitude. Don't rely on the accuracy of anything an LLM gives you without verifying it in some way. And the second thing is that this has been called the part of the jagged frontier of AI which is. It's a very strange thing that a large language model to certain things incredibly well and quickly. Again I'll say summarizing text, rephrasing text in different ways, whether it be everyone I think knows now explain the following to me like I'm a five-year-old, or explain this to me like I'm an expert, put this in a humorous tone or something. I mean. Llm tend to be incredibly good at that and yet they're really really bad at some other things. So that's the jagged frontier I often picture in my mind. I have an image of a guy who goes to the gym and only works on his arms right and he's got these huge biceps and tiny little scrawny legs. That's kind of what an LLM is like. But the output doesn't give you a clue that that's what's going on. Every answer you get from chatGPT chatGPT sort of portrays as if it's equally good. So that's again why it's a strange situation where the tool itself or its output doesn't give you any indication of what its quality is. So that's why I understand and validate the criticisms of hallucinations and so forth. But what I don't agree with is then jumping to the conclusion that you should never use the tools. I think a wiser, more productive conclusion is learn what the strengths and weaknesses are, so that you can either choose when to use certain tools and when not to use them, and when and how to massage or error correct or improve upon the outputs. And given the incredible speed I mean. Look, and the unstated assumption we're talking about is whether it be an LLM or other kinds of AI you can produce outputs that would have taken a human a thousand times longer to produce, and maybe not even produce output. That was good. When you're dealing with that kind of speed and cost amplification, you have to take seriously the value of it and at least look into how can I retain most of those benefits by coupling it with some on the input side or output side correction to still get a very, very significant improvement in quality, speed and reduction in cost. 0:45:01 - Mehmet And one thing I want to add, robert, and this is back to something you mentioned a couple of minutes back. It's regarding us humans. We want to be lazy. Actually, let's face it, humans, by nature, the brain give always the commands to search for shortcuts. And when this is something even I learned by practice when I start to use these tools, because when I was thinking, okay, that AI can do everything for me, and then I figured out, I did a couple of mistakes myself and said, hey, hold on, I need to check on the work of AI. Yeah, it's not 100% accurate, but actually I can say, at least for me, 90% of the time it was accurate. And even I start to share with everyone, like guys, look, it's not bad idea to go, for example, write a marketing material and email, copywriting, whatever, but when you copy and paste it, check it before, right, so at least go and read it. You need to still do that. And the second thing, because what you mentioned, robert, reminded me of when Wikipedia first appeared, right so? And everyone saying okay, like, yeah, like let's use Wikipedia, and people start later on they say, okay, but you need to go check their sources, because this is something it's a collaborative work of many contributors, and maybe some people will have bias, and here I can relate to the AI, llm and the bias data and all this. So, like any other technology, we don't need to assume 100% that we okay, we can just give it the word and it will do that for us. And I think this is very valid point and the way how we can enhance it to your point. And I think even OpenAI and even Google, they have this capability within the interface. If you like it, you have the sum up, if you disagree, you have the sum down, and even you can write some comments, and I know, at least in the enterprise edition of chat, gpt like, even you can customize the LLM the way you want and even you can give the instruction how you want it. Even you can do it if you have the plus, so I can go and say, hey, my name is Mehmet, this is what I do, this is what I live. So every time I ask something, it takes these instructions and it knows you know what I'm trying to do. So it's start to learn. So this is the feedback loop to your point, robert. It's very important. And people, yeah, they should not be lazy too much, you know, relying 100%, of course, and this is why, like any other technology, like you know, like this is also remind me of one of my guests who mentioned about, for example, the autopilot of a car, right, the self-driving car. Like, at some stages even they tell you in such situation, don't enable the self-driving because you need to be in control. So this is the same thing. Now, one final thing, as we approached Vanna and Robert and I love this, but, you know, let's try to hear it from you also as well it's about learning from history, and in the article you mentioned about, you know, blaise Pascal and the evolution of mechanical calculators. So and you did this, you know, I would say analogy of this. So what are the lessons from the history of technological advancements that you believe are particularly relevant to the current discourse of the AI? 0:48:42 - Robert Yeah, I mean this is where we get a little bit philosophical and introspective, but I think it's very important. The code I used from Blaise Pascal when he invented the first mechanical calculator. It was 1640 or so, so we're talking about, you know, 400 years ago. And what he said, and I'm not gonna remember it exactly, but he said I hope that this invention, you know, which can perform arithmetic using gears and levers, will finally put to rest this misunderstanding or bias that we humans have for believing that that type of mental activity can only be performed by us and can't be. I think the English translation I had was something like those mental operations can't be consigned to a physical apparatus that can perform the same functions in a different way. And so I suspect at that time he was dealing with the objection from people that, hey, you can't take mathematics, which is an inherently human endeavor, and put it into a machine. A machine can't think, a machine can't reason. And this is mathematics, is inherently something that involves thinking and reasoning and is something that is uniquely human. Those are exactly the same things people are saying today. Look at all the things that computers have been able to do, often in radically different ways than humans. Do them, but do them nonetheless. You know. That proves Pascal's point that often, mechanically, you can perform a function that achieves the same result as a human would have, but in a radically different way. I mean, you mentioned chess, right? Computer chess programs don't really play chess in the same way as humans do, but they perform the same result. In the history of engineering, people often point to the airplane. For many years people were trying to build this is before the Wright brothers. How were people trying to build airplanes? They were looking at birds and they were trying to build mechanical devices that took off by flapping their wings. And then the Wright brothers came along and realized you could achieve the same result using a different physical mechanism than we knew about before. This happens on and on again, but for some reason humans are drawn to either one trying to believing that machines can only perform the same functions as we perform in our minds, by doing those functions in the same way that we do that's one misconception and therefore by trying to design systems that don't just achieve the same results as us but that mimic our own way of doing it, and those often fail right. And then something comes along like a large language model which I think actually doesn't produce text in a way that's very similar to the way the human mind does it. That's the approach that ended up being necessary to perform a similar function to us but in a very different way. But chess is another example. You can go on and on and on. So that's one belief, that it is necessary that in order to get the same results you have to mimic a human mental process. So that's often incorrect. The second thing is the belief that if a machine is producing output that looks like human output but doesn't do it in the same way, it can't be useful. There are two slip sides of the same coin. And yet we've seen over and over again that it can. But we're running up against that, particularly that second belief, a lot right now, and I think the proof is in the pudding. We don't have to engage really in debates over how LLMs are doing what they're doing, whether they're doing things the same way as us. I mean the philosophical debate about whether human type reasoning is necessary to solve certain types of problems is an interesting one. But if people keep improving the technology and it just demonstrably is able to solve problems and achieve real world tasks, that's what's going to push things forward, regardless of whether the technology does achieves those results in the same way as we do 100% and you know, like, again, I don't like to whether it's achievable, we think it's doable, not doable. 0:53:30 - Mehmet I would leave this to the flow of the events. I would say because, if I'm sure you know and you talked about the AI, winter, but which is what? The very long back, but I'm sure that if you have asked someone, maybe one month or two months before charge EPD appeared, something like this can happen. Probably they'll tell you. You know, maybe we still need 10 years, 15 years, maybe it will never happen, right. So the only thing and this again to your point, some stuff is philosophical a little bit, but what if I had anything? You know, during my career, and you know the span of my observation of technology, you never say never, right, like we don't know where things can be heading and everything is possible. The only thing which is, I believe it's true is things are accelerating really, really fast. So I think you know, like someone just pulled the plug and you know everything started now to go very fast and I think no one can stop it. If you remember, robert Lossy, there was some voices to slow down all these things, of course, and I found it. I remember I was still doing some solo episodes and I was saying, like, come on, guys, like this is nonsense, because if the big guys they stop, this technology is already out, it's in the wild. The LLMs they became, some of them open sourced, you know, the technology became very democratized that you can't stop it anymore. Like if Microsoft open AI, google, I don't know, like all these big companies, they don't do it, but the bad guys gonna start to do it and they're gonna do it. So better we stay, you know, on the edge of the technology and keep doing it. So this is my two cents at the end. So, robert, like, really, can you remind us where we can find more about you before we close? 0:55:31 - Robert Yeah, thanks so much. I'll just close to just to chime in on what you said you know about just seeing where the flow goes. You know, I think this is a time in which we all would benefit from open-mindedness. Be open to your own biases or preconceived conclusions being wrong, perhaps being really curious and humble. You know, if you combine all and have a willingness to learn, if you combine all those things together, I think we can just move past a lot of these debates about what AI can or can't do. We'll see what it can do. As you said, that's to see where things are. We're gonna see. None of us can answer in advance what it can or can't do, and none of us can answer whether it's gonna be able to replace it. We have to just be open to new information as it comes to us, be open-minded, curious and be willing to learn. So thanks for letting me get on the soapbox for a minute In terms of where people can find me. If you're interested in everything I'm writing about in regards to skills that you need to leverage and take maximum benefit of AI, go to robertquokkencom. You can always follow me on LinkedIn. I post there really regularly, linkedincom, slash in slash Robert Quokken and on, I think, what we talked about more last time, the patenting of AI and patenting of the software side of things. You go to my law firm at blueshiftipcom. 0:56:54 - Mehmet Thank you very much, robert, and for the lessons. You can go check all the links in the show notes. You will find them there. Thank you again, robert, for this very insightful discussion. Again, I enjoyed the conversation, like always, and I'm sure the audience also will love it, and this is how we end every episode. So for the people who that just discovered this podcast for the first time, thank you for passing by. I hope you enjoyed it and I appreciate if you can give us a thumb up and also to subscribe who are available or all podcasting platform Apple Podcasts, spotify and all the rest Google Podcasts and so on and if you are one of the loyal followers. Thank you very much for all your support and for your loyalty. I appreciate that. Keep your feedback coming. Keep your reviews coming, even if you don't like anything, you can just try this. I love also to read, like you know, feedbacks about something I should enhance, right, so keep them coming. And also, if you're interested to be on the show, don't hesitate to reach out. You know where to find me and hope to meet again in a new episode very soon. Thank you, bye-bye.