#332 The Role of AI in Streamlining Supply Chain Processes: Insights from TrueGradient Cofounders
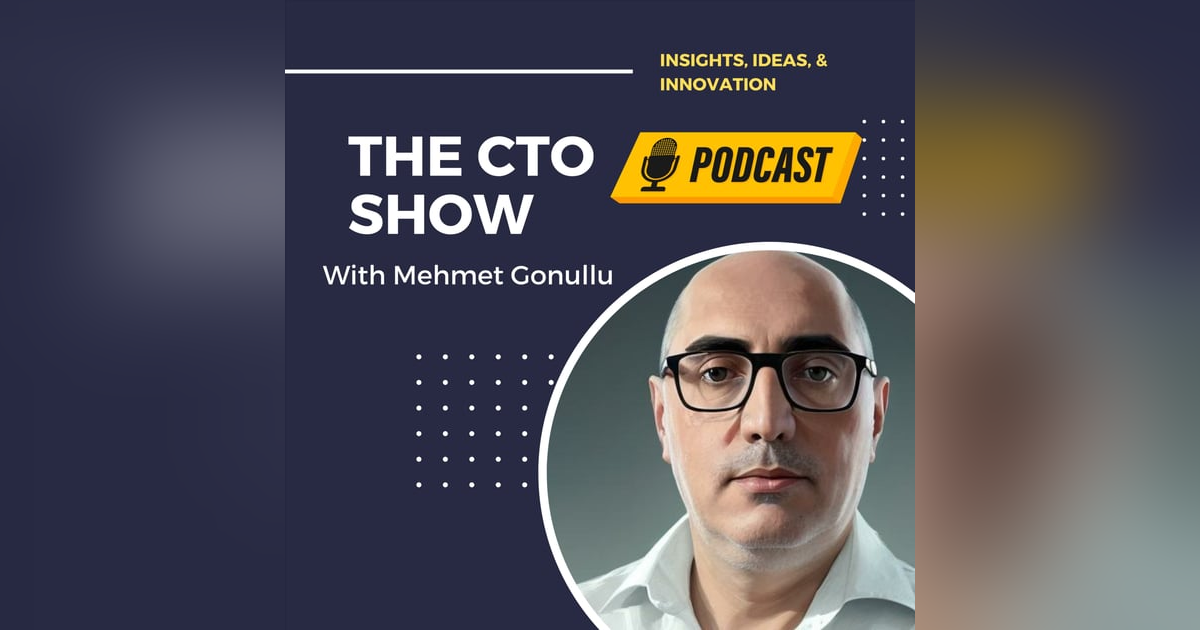
Join us on this enlightening episode of "The CTO Show with Mehmet" as we delve into the transformative world of AI-driven supply chain management with the cofounders of TrueGradient, CEO Ankur Verma and CRO Jasneet Kohli. Discover how True Gradient's self-serve AI platform is revolutionizing demand forecasting, inventory optimization, and price optimization in the CPG and retail sectors. Learn about the challenges and solutions in implementing AI technologies directly from the visionaries behind one of the industry's leading innovations.
What You Will Learn:
- The concept and benefits of self-serve AI in supply chain management.
- Key challenges businesses face in supply chain optimization and how AI can address them.
- Practical insights into deploying AI solutions in real-world business scenarios.
- The future of AI in improving business decision-making and operational efficiency.
Find More about TrueGradient here:
01:17 Introducing True Gradient: Revolutionizing Supply Chain with AI
02:27 Exploring Self-Serve AI in Supply Chain Planning
04:24 Business Impact: Solving Real-World Problems with AI
09:01 Technical Innovations Behind True Gradient AI
14:26 Quantifying the Benefits: True Gradient AI in Numbers
17:52 Enhancing Decision Making in Supply Chain with AI Technology
24:23 Overcoming Implementation Challenges of AI in Business
31:36 Strategies for Successful AI Adoption and Implementation
38:50 Closing Thoughts and Future Directions
[00:00:00]
Mehmet: Hello and welcome back to a new episode of the CTO Show with Mehmet. This is maybe the third time I'm doing, I'm having again a guest that I had last year, but this time bringing another co founder with him. So Ankur Verma, thank you very much for [00:01:00] being here. Co founder of True Gradient and we have Jasneet
Mehmet: he's also a co founder and chief revenue officer. It's good to see you again, Ankur and Jasneet you know, thank you for joining Ankur this time because we're gonna tackle from both maybe technology and business perspective. So just quickly, uh, if I'm not sure, Ankur, maybe just remind us, you know, what true gradient is and what you do guys.
Mehmet: And then we can start to take it from there.
Ankur: Absolutely. Thank you, Mehmet. So it's my pleasure to be on on on the show again. Uh, thank you so much for hosting me again over here along with JK Jasneet over here. Uh, amazing. Uh, yeah, real quick about True Gradient AI. So this company is, uh, is a self serve AI platform, which has been built for supply chain optimization, family built for solving problems like demand forecasting, inventory optimization, price optimization, assortment.
Ankur: Uh in the cpg retail [00:02:00] world, uh today there are problems related to excess stock stockouts Uh, some of the pricing related issues. So supply chain optimization platform Is a platform which really eases for any supply chain analyst or somebody who's not very well versed with a technology, machine learning technology or deep learning technology can go ahead and use this to solve different problems in their area.
Ankur: Coming
Mehmet: back again, Ankur. Thank you for that. Now, like for someone who might not be familiar because you mentioned a couple of things, but I'm interested to know in what is the self serve AI in supply chain planning? Can you like a little bit deep dive more into it?
Ankur: Absolutely. That's right. Uh, so, uh, The self serve AI, which we talk about it, I would say it's a really a new category that we are trying to build out over here.
Ankur: And the reason for that is, uh, in all over the places, whenever we talk about any e commerce company, any [00:03:00] retailer, CPGs, so over there, there are certain places, certain supply chain analysts who are taking decisions day in and day out, right? And all of them is all of they are working primarily on Excel sheets.
Ankur: And by trying to solve problems through some of the approaches which are, uh, which are very smart, but not a ML driven or AI driven, uh, approaches. That is being worked over here. What we did is we're trying to create, we have built this platform, which can be accessible to any supply chain analyst or any analyst for that matter.
Ankur: They can come, uh, connect the data, right. And build models over it to be able to solve different types of problems in a self serve manner. So we onboard them to a training session and then let them or allow them to use it. For any time and every time they want to generate any type of solution that can be related to a demand forecasting Inventory optimization as I mentioned during the introduction of true gradient AI Over here.
Ankur: So that's mainly what we are [00:04:00] calling self serve AI and and and primarily the most important thing being the Neuro optimization models or the machine learning and deep learning models, which are today tackled by like the neuroscience folks or the data scientists who are sitting across the globe has become accessible to these supply chain analysts through the true gradient AI in a sales of manner.
Ankur: That's great.
Mehmet: Yeah, absolutely. Absolutely. But now let's shift to more the business side of the story and, uh, just need like you, you take care of, of the, uh, sales organization. And of course, you know, when you talk to your customers, you need to talk about the different use cases or maybe the business problems that your platform and such platforms can solve.
Mehmet: So if you can little bit tell us what are the problems or the challenges that businesses are facing and you know, how you, you know, you convey the message to them from a use case [00:05:00] perspective.
Jasneet: Right. Thanks, Mehmet. Uh, uh, great to be here. And, uh, you know, and great question. So, you know, there are various use cases or problems that can be solved, uh, to, uh, self serve, uh, artificial intelligence paradigm.
Jasneet: Uh, right. I think, but the most valuable in our opinion, and when you say most valuable, you mean those problems, uh, which move that, you know, uh, right. Which basically, uh, move the dial a lot for the company, uh, demand forecasting, uh, inventory optimization, promotion optimization. I think, you know, kind of alluded to that in the beginning, but, you know, the business context is in particular CPG and retail, uh, you know, the leading indicator of supply chain health for a retailer is basically on shelf availability.
Jasneet: For example, if you walk into a Walmart store and [00:06:00] you're looking for a product, and if it is not there, you're disappointed, right? And so, you know, out of stock situation and not only it is loss of sales, uh, for Walmart, but it is, it might be also loss of customer because next time you might go to some other retail.
Jasneet: So it's a very big, and it all relates to, I mean, if the on chip availability is poor, uh, it is. Mainly driven by, uh, you know, factors like, you know, uh, not able to predict the demand of the consumer accurately and not able to also have the right, uh, optimal, I call it, not right, I call it optimal is technically the right word, optimal inventory mix.
Jasneet: Uh in the network in the supply chain network in particularly store, but even upstream supply chain locations, right? so, uh Will that and that is that is one of the things again is solved by forecasting and inventory optimization Uh, and then for on the other hand, uh the [00:07:00] mandate or one of the important api for cpg Because you know product good company is fill rate, which is their you know, which measures their ability to To fulfill orders coming from a retailer.
Jasneet: Uh, for example, a Mondelez fulfilling, uh, you know, Wal Mart order will be measured on the airfare rate. So again, you know, for them as well to be able to accurately predict the demand off an end consumer like how could I have yourself is is super critical. And then an optimal inventory profile along with that, retailers and GPG companies offer discounts, promotions.
Jasneet: What is the right promotion depth? Right? I mean, if you walk into, uh, you know, let's say a Nike store or an Adidas store and you see a percentage discount of 20%, 30%, uh, you know, you, you, you know, there is science and math behind coming up with that number, right? How do you come up with the optimal number?
Jasneet: Uh, you know, you don't want to give a big, very big discount and you know, which leads [00:08:00] to margin erosion. Right. But you wanna just meet the right optimal number, uh, to meet your sell through target, uh, within the timeline that you have. So that is called kind of optimal. So these are the big three large problems you're solving at the end of the day.
Jasneet: Uh, ensuring, you know, the age old, uh, you know, phrase, which is always used in the supply chain world. Uh, you know, uh, having the uh, right inventory. at the right time in the right quantity, right for CPG and retail.
Mehmet: That's great. And of course, you know, like this is all to, you know, it goes to, to, to increasing efficiency, it goes to increasing revenues and avoiding, avoiding risks of overstock or low stocks and all these things.
Mehmet: Now, you mentioned like some, some. You know, uh, features or let's say some elements that, uh, are in the self serve [00:09:00] AI, uh, minutes ago. So you mentioned machine learning and, you know, the others, but like, what are like some other features, uh, from technology perspective, uh, for having, you know, like a proper self service AI in supply chain planning?
Ankur: That's a great question, Mehmet. And, and this is something which is, I would like to actually elaborately answer this question because Bringing up True Gradient AI, the kind of features that we brought in was through our entire experience. So all four founders who are part of True Gradient, in the past six years to seven years, one of the things which, I mean, I also had faced challenge and a lot of other data science folks and analysts faced this challenge is to be able to get an interface, right, as an example.
Ankur: Right. If I have to run certain deep learning models or some of the models which are complicated enough and this requires certain infrastructure, there doesn't exist any very sweet interface wherein I can [00:10:00] go, tag the data, call up these complicated neural network or machine learning algorithms and run, run through it.
Ankur: So what we wanted to create is rather than going to every, like, whenever a new project comes, rather than coding it right from scratch, There should be a capability, a very user friendly capability, which can help any analyst, any data scientists to use that and deploy their models. So the first thing which I will call is the user interface, very deep, very, very connected to any analyst who is very much acquainted to work into such.
Ankur: That's the first thing which we wanted to bring together. It took a lot of thought, really two to three years of thought, years of thought process to how do we simplify this process because it's not an easy problem to solve. The second one, we realized that data engineering is a, is, is, is giant. That is one of the biggest problems that a lot of people, like whenever we talk to a customer, the first question which comes, hey, are you solving your data problem?
Ankur: Like, is the data integration seamless? Are we able to connect to different types of connectors, [00:11:00] uh, within a shorter period of time? So that is the second problem wherein I talk about having multiple connectors into the system, API level connections. So that we can connect different systems and bring the data together into true gradient so that none of the insights really gets missed while we are building any machine learning models or deep learning models.
Ankur: So that's the second part, which is around the data connected part. And then, yeah, then, then it's all about how good your models are. Can you, can you extract multiple features or factorization? Uh, just to give you an example, we work with certain customers, uh, who let's say Ramadan is going on at this point of time, right?
Ankur: So, and it is a moving season. So for a, for a FMCG brand, for a food and beverage brand, it's super critical to understand how is the demand shifting happening over there. So in order to accomplish that, our AI models are capable enough to generate the right factors to learn and generate the right factors and do the predictions accordingly.
Ankur: So. [00:12:00] That's an, and we also use a bit of generative AI technique over there to be able to generate, uh, uh, the factors or the levers which will be, which will make our predictability better. So that's the machine learning and deep learning part of it, which is around the predictions and all. Uh, then. One of the important thing is optimization once you build the models.
Ankur: So because as JK was mentioning, from business standpoint, what matters to business is are we reducing the stockouts? Are we optimizing the inventory to not to get into a waste stage kind of scenario. Are we getting the price prices right or not? So that requires optimization, deep optimization methodologies.
Ankur: And every business is unique, as we all know. So what we are able to do through Trigradient is to be able to add multiple constraints. So as an example, we work with a couple of customers wherein we are having as much as like 20 constraints, be it supply side constraints, demand side constraints, to be able to run, [00:13:00] uh, the inventory optimization, because there are these many constraints for them to be able to, uh, be predictable, uh, for, for them.
Ankur: So that's the optimization bit which we have brought into our system. Final bit is around the once you have built the forecast, uh, it might not suffice well because again, uh, there can be some of the unpredictables, right? So that what we allow is scenario planning activity, wherein we allow the supply chain analyst or a planner to go ahead, make certain enrichments.
Ankur: Yeah. Like, I mean, I was, uh, during the COVID time I was doing forecasting at that point of time as well. It was it was really a hard time to forecast because if the data is lost if there is no data for a given point of time you need to bank upon some of the adjustments or the enrichments that you do.
Ankur: So our platform allows to do different types of enrichments to change different types of scenarios and rebuild the Rebuild or enrich your forecast or the optimization basis that [00:14:00] so yeah long answer But yeah, I just wanted to bring all the aspects together over here. No,
Mehmet: that's that's perfectly Explained and thank you Ankur for you know, this detailed explanation.
Mehmet: So the aim here is also to educate Also, you know, on technical level, how these things and why these things are important that we just did this, um, like now I would go back to just need, and you know, we, you mentioned a little bit about the use cases. Now, if you, you want to put some numbers next to, to these benefits.
Mehmet: So how, how does, you know, the self service AI, you know, from numbers perspective, benefit supply chain organizations?
Jasneet: Yup. Yup. Yup. No, that's a great question. Again, I think. See, going back to what I mentioned, let's start from there. Let's start the peeling. Let's start peeling the onion kind of from that broad objective.
Jasneet: Any organization that exists, a retailer or a CPG to service a customer, a consumer, right? Especially the retailer. [00:15:00] So it all starts from a service level. Everybody wants to be best in class in terms of your service level. Um, And, uh, in that service level, basically, first of all, then helps you grow your revenue and margin because reduces your losses.
Jasneet: So we think that best in class, uh, supply chains, uh, you know, can actually then use, uh, these levers, uh, you know, uh, to improve. So to improve your service level, grow your revenue and grow your margin, you know, uh, uh, sophisticated, get there. Cells of a methodology, which just not on the said, which involves, uh, you know, very sophisticated forecasting techniques and optimization techniques can help forecast accuracy to go up by double digit.
Jasneet: Almost. We have seen that in some cases we have seen almost 20 percent improvement in forecasting. This is that is dramatic, right? And that happens, [00:16:00] uh, especially. For mid enterprise sector, uh, you know, unfortunately that sector is totally, uh, doesn't have access to artificial intelligence or machine learning, the investment which large companies does.
Jasneet: That is also kind of our mission, right? To democratize, uh, artificial intelligence for companies, uh, who don't have access to sophisticated technology or sophisticated modeling techniques, let's say. Uh, and yeah, so double digit forecast accuracy improvement. And then the second is inventory optimization.
Jasneet: Uh, one of the metric where what measured is days of supply. So, you know, up to 30 percent adoption in, in days of supply, uh, is what we have seen And, and the last is I think the markdown spend or promotion spend. So like I mentioned, sometimes you're just overspending on promotions for markdown and without any, any benefit, right?
Jasneet: A 10 percent markdown might just deliver the same outcome rather than a 40 percent markdown [00:17:00] for instance, right? Okay, so you could have been just 10 percent to kind of get rid of your inventory in 30 days, for instance, and but you end up giving higher because you don't have, uh, the right science or math model behind those decisions.
Jasneet: So, uh, those are the three broad, uh, kind of areas and the results which we have seen or we have seen in our past experience and, and thankfully, companies, large, small or mid sized who are. Embracing, uh, this fast evolving, uh, concept or a paradigm are able to realize, uh, I believe.
Mehmet: That's great to hear Justin, of course, like this is, uh, I like to hear these numbers and these stories because, you know, they, they resonate also with the, with decision makers and mentioning decision makers because decision makers, they take decisions, right?
Mehmet: So I have this question for you both and like, feel free, who would answer me this first, maybe Ankur, you would answer [00:18:00] maybe more from a technical perspective and just that you would answer from business perspective. So how, how the technology enhanced the decision making actually in, in supply chain planning?
Ankur: No, that's
Jasneet: great. Why don't we go ahead first, Ankur? Yeah,
Ankur: sure.
Jasneet: Sure, JK.
Ankur: Yeah. One of the thing that, uh, me and JK, we always talk about, right? Since past six months, we have been talking about to get numbers on the tip, like on the tip of the supply chain, they should have, you know, which are the items which are going out of stock.
Ankur: What are the items which are in excess over there? So from the product perspective, what we enable our customers is to feed them all the data that they are looking at. Every day. Yeah. So there are decisions for, let's say if we talk about the F& B category, personal care category. So these types of categories that decision are being taken maybe at a day part level, like every four hours there is a replenishment required for certain items.
Ankur: Right. So [00:19:00] we, our product like True Gradient AI enables the supply chain analyst and these decision makers to be able to proactively take these decisions, yeah, from the, from our predictive modeling power that we have generated. So they are able to know beforehand that what are those 10 items which we need to restock for the next day or for the next few hours so that they don't go out of stock.
Ankur: So that is a very critical or crucial thing that we see, uh, from decision making perspective. The second thing is also about a lot of aging and wastage problem is prevalent in the FMCG industry now. So once we see that there are certain items which are not going cut, not, not going to cut through the market, uh, because they might expire or the tolerance might be too high for the retailers to take it, uh, then our platform really.
Ankur: Allows the user to take a quick decision to take a call around if they have to make a pricing decision, then what is the pricing decision that they need to take to our optimization systems at the price [00:20:00] recommendation optimization systems. So what we try to build is entire interconnected AI. So right from the demand forecasting to inventory to pricing to assortment.
Ankur: There is a single flow which flows and every module knows what's going on with the other world Over there. So that's my take over over over the decision making enablement and anything you
Mehmet: yeah great Ankur Justin anything you want to add maybe from a different? Yeah.
Jasneet: Yeah. No, I think I think I can have I can I can add the business perspective here I mean, let's talk about CPG, right?
Jasneet: I mean, a CPG company, I like to break it into three kind of horizons. Uh, one is strategic horizon, strategic planning, and second is midterm planning. I call it SNOP planning. And third is. I call it the execution horizon where the sensing and replenishment happens. So if I break it down, you know, the prediction, which I mentioned about, it can be in short term horizon, midterm and long term.[00:21:00]
Jasneet: So a long term horizon prediction or forecast, let's say, which is, you know, which is like five years of forecast, right? It can be used for strategic decisions. For example, I'm a CPG company. And I have to decide where to shut the plant, where to open the plant, where should I invest in new warehouses, which markets I should go, right?
Jasneet: Those kinds of decisions are based upon five years of what we call, called, uh, capacity planning or network design decisions, right? Uh, so that is one, uh, very important https: otter. ai
Jasneet: Very well known term in industry sales and operation planning, or even called integrated business planning, where you know, you are providing forecast in weeks and months bucket, and that forecast is used to procure material or is used to also produce material, let's say, and then you, you know, then you are [00:22:00] placing the inventory in the right, uh, right place.
Jasneet: So that you're able to service your retailer, but from C2G perspective, so that's called sales and finally is, you know, the execution horizon where you are moving products really from the distribution center to, uh, the retail store, right? And that's called execution. And we use sensing algorithms daily level insight, right?
Jasneet: Well, that's the C2G piece, right? So it's the full spectrum, right? At the end of the day, demand forecast is the backbone of all the C2G. You can think of it as a unified demand forecasting to do in different horizons, right? And if I go to the retail side of the story, uh, it's a bit different, uh, right? But in retail, actually, you can go very different for grocery and it can be very different for fashion.
Jasneet: Uh, but perhaps quickly talking about just fashion space, uh, is that you have pre season planning and you have in season planning. Then again, you know, pre [00:23:00] season means that if I am Uh, planning, let's say for, uh, you know, the spring fashion, uh, lines that I have, you know, you know, folks in us, uh, on North America have to plan it more than 12 months in advance because some of the supplies are coming from Asia, uh, long, so you use long term forecast again.
Jasneet: Uh, to kind of, you know, giving your orders to your vendors, uh, you know, for, for the season. So that's preseason. Then there is an in season piece, you know, you have all your product in a distribution center. Uh, let's say imagine, and then you want to then, uh, allocate, uh, that product different stores in different States.
Jasneet: Uh, right. And then that's where it becomes more like a close to an execution use case. So those are the kind of business processes, if you will, which get impacted by, uh, the underlying science and tech.
Mehmet: That's great. Yeah, absolutely. And it's a [00:24:00] great, um, I would say, you know, complete picture, I would call it and complete perspective on, uh, on that aspect now, of course, you know, and this is a question.
Mehmet: Uh, in technology is always asked, and I think it's none different than with self serve AI. Anytime we want to implement a technology, so people say, okay, talking about it in, on, on the slide and, you know, in, in, uh, You know, the best case scenario is something and when it comes to implementation and putting it in action is something else and question for both of you.
Mehmet: Maybe I could, you could, you could tackle from, uh, technology perspective and just from maybe business perspective. What are usually the challenges? That you've seen associated with implementing this technology?
Ankur: Yeah, I think that's a, that's a burning question. , [00:25:00] mammoth and, uh, and, and, and, yeah, this is, this is a question which actually I get a lot of times when I talk to, whenever I present, when I pitch to a larger, especially a.
Ankur: larger enterprise or larger companies. And, and, and I distribute, I say that there are four aspects to, to the, to the challenges that we face out over here. Uh, the first one being the data quality itself, like there's a lot of data for us to get excellent results using some machine learning methodologies.
Ankur: It cannot be a garbage in garbage out. So it's very, very important that we do a lot of due diligence over the data. Itself, which is being flown into the system so that we make sure that the decision making is accurate, right? We're, we're looking at the right data for the accurate decision making. So data quality becomes the first aspect of the entire framework that we look at.
Ankur: Second comes when, when we want to, when we want to really solve these problems. So it cannot be because you're talking about [00:26:00] massive data flowing into it. So it has to be integrated. I was talking about some of the features that we built in into our framework. Obviously, there are hundreds and thousands of, uh, uh, like ERP systems.
Ankur: There are so many, uh, like, uh, WMS systems, which is sitting out there. Different customers have different types of places where the data resides. So integration bit is the second bit for the implementation perspective. That is our product seamlessly integrated to the respective ERP or not. So that's the second bit that we try to ensure and the challenge which comes to us.
Ankur: For solving it. The third point comes a lot around the security aspect because it is a very important, I mean, there are government laws. I mean, just now a new law came in the European from the European laws that we saw, right? So there's a lot of attention, which is, which is being paid to be able to get the data intact too, so that, uh, so that the data is not being used otherwise, or, you know, [00:27:00] uh, it has to be used in a manner that it needs to be implement for implementation purpose.
Ankur: And the fourth one, which is the largest one for us. Once you have cracked a customer and you are working with adoption, the adoption is the fourth one. Wherein, how do you make sure that, uh, the business, which has been operating in a particular way since past 20 years, or maybe 10 years or five years, whatever that timeframe is, uh, new technology that we are bringing into the system is able to make that difference.
Ankur: Yeah. Is able to be utilized and, uh, the supply chain managers, the I'm using it well. So yeah, that's that's my four points
Mehmet: It's it's it's a complete 360 degree. I would say of all the challenges. So just that you want to add something on that Maybe you see from a different perspective
Jasneet: Yeah, I think uh, you know, that was definitely a very comprehensive uh, you know, uh Outlook on the challenges one thing from business point of view, which I think, uh, you know all the projects uh ai projects i've been involved in [00:28:00] Is, uh, you know, challenge or opportunity.
Jasneet: You can say is that, uh, you know, sometimes I also has, uh, it needs a lot of buy in from the top management articulation of vision. What is really going to do? How it is going to empower? The workforce and, you know, not a negative way, but in a good way, right? Because at the end of the day, you know, these tools are able to unlock productivity.
Jasneet: And I believe humans can work on more high value work in the firefighting and more of a, you know, what can be given to the machines, right? So that vision and that plan has to be communicated. Uh, very well. Uh, and you need to reiterate that from the top management perspective. Uh, so that the change management piece is easy.
Jasneet: And another thing I think the challenge involved is also identifying the right champion, [00:29:00] uh, right within the organization who can work on the project, right? Who can ensure, you know, understands us all and able to kind of be the catalyst of change. Do identify that champion is super, super critical, right?
Jasneet: I really believe if you have the right side of champions working together in collaboration with technology providers and all that can make a huge difference. I have seen so many times more talented teams and create solution, not flying just because there was no alignment on the team was on the ground working right?
Jasneet: So that is very important. I guess.
Mehmet: And, you know, my two cents into what you just mentioned, Jasneet, and of course, Ankur, all from technical point of view, absolutely. You know, and privacy is, is key by the way, and, uh, we're going to have very soon a, a, a complete episode with a guest on, on, on data privacy and, you know, all the aspect of that [00:30:00] related to AI specifically, but to just this point, I believe, you know, uh, it should, to your point, it should come from top, you know, it should be, you know, A plan from the executive levels.
Mehmet: I think we started to see the light at the end of the tunnel, because unfortunately, and I'm saying this, people might agree or not agree with me. We've been at corporate level all the time, reactive to the technology, because we've seen someone is implementing it or someone heard something, you know, we implement this, the things start from self assessing the current situation.
Mehmet: And similar to, to, because. Of course, what you do is something more advanced than just a digital transformation kind of thing. You know, uh, initiative. It's something that can take the business to a complete different level. I'm talking here about, of course, the sectors that you must, uh, interact with, which is, you know, retail, supply chain and everything in between.
Mehmet: So this have to come [00:31:00] from up there, of course. And, you know, to your point, of course, you need someone who would be the champion if we can. Yeah. This way, of course, definitely people in sales will understand more about this, but I mean, from business perspective, so a champion for whoever initiated this initiative from the board level.
Mehmet: So someone who can take this and, you know, go to the organization, talk to every single one and convince them that, Hey guys, we need to implement this. And this is, you know, I'm relating this to the question. We mentioned the challenges. But the question next, which is very natural, how we overcome with these challenges.
Mehmet: So I want to hear from, from both of you on this, um, Ankur and Jasneet so from technical aspect, Ankur, you mentioned a lot of things. How, how are you tackling or how organizations are tackling these, these challenges?
Ankur: Absolutely. And I think JK and yourself just now mentioned about getting a champion, right?
Ankur: Within the team. And that plays one of the most [00:32:00] crucial role in addressing. A few of the challenges because uh out of four Challenges that I mentioned one of the problem is mainly related to how good the quality of data is Are we able to integrate the system? These are the two points which I talked about, right?
Ankur: So it becomes really important to get someone in the team a hero in the team who can help us to, uh, to do a lot of validation, right? Someone who is believing in what we are doing over here. Yeah. It is making more importantly, it is making the product is making his or her life easier. And then the attention span comes out there automatically.
Ankur: Right. And that helps us to do a very strong validation of different data, which is flowing. And really, I mean, practically speaking, it happens for like, One to two cycles by like two months. We are doing a lot of very intense validation over what was already prevalent and what true gradient is providing just from the input data perspective and what we are seeing as an output.
Ankur: So that, that, that, that addresses the first second one, [00:33:00] uh, which is, which, which is, which is fundamentally, uh, how all of Has evolved over the time is training. So having a very, uh, intuitive, intuitive training materials, right. Which can be understood by anyone who wants to use the system is what our focus has been, and we have seen a lot of progress in past six months by getting those materials out, getting very, uh, you know, like, uh, very, very sharp.
Ankur: Sharp commentaries about different features that are there in the model or in the, uh, in the platform itself. So these are the two main aspects, which, which, which, which works primarily for us from that perspective. And, uh, from the security standpoint, uh, I would say we are working on that because, uh, data governance law abiding by all the data governance laws that we just are different compliances, which new you need to do.
Ankur: Uh, as a platform, as a SaaS service. So we are putting a lot of, [00:34:00] we are investing and we are putting a lot of effort to ensure that when we are talking to customers, we can show the certificates to them that we are at the top of it. And, uh, and the data is extremely secure because that is number one priority for us as well.
Ankur: So that's my, uh, that's my two cents on that. Yeah. Thank you.
Jasneet: Yeah. Excellent. Excellent. No, thanks. That was good. I think. I think I'll add to two or three points here. One is, uh, you know, both from technical and, and, and business perspective. Now, I think one thing which I have, uh, you know, seen again in past experience, which really has, especially on data quality is upfront coming up with a data quality mitigation plant, uh, with your customer.
Jasneet: As in, for instance, you've been in situations where customer would say, okay, uh, you know, and then there's always, you know, There is never, uh, what I call is, uh, you know, uh, a perfect state, uh, of good data. Even very large enterprises are struggling with, [00:35:00] uh, you know, quality data quality challenges, right?
Jasneet: So it's always important to know what is mandatory data and what is good to have data. That perspective should be here. And then especially on the good to have data, you know, what do you have right now and what are the methodologies to. Overcome that, uh, gaps in a gas over a period of time, for instance, you know, one very tangible example is some customers would say that I have, I don't, I don't have point of sales data for all my retailers, right?
Jasneet: Normally they have for large retailers give point of sales, but then you can use shipment data as a proxy. To start the journey and then you can also set up timelines with your customer. Okay, you know, maybe one year down the line These customers you should try to acquire points Right. Because if you get policy as data, by the way, you know, you would get this much accuracy gain.
Jasneet: And then you can do the analysis and it is worth the investment to acquire the data. Those kind of [00:36:00] objective discussions should start happening on data mitigation plan. Second thing I like I alluded, I think, you know, identifying the champion and chain management You know, building communication plans from the top and having also investing some, uh, you know, focus on investing in chain management program is important, right?
Jasneet: Upfront. Uh, you know, I have seen companies who take it seriously. They will come up with, you know, what is the communication plan, some newsletters and conversations from the top management, right? Ensuring the typical, you know, proper steering committee sponsors. Uh, but more importantly, I think, you know, given the champion, we were talking about a platform to socialize what he or she is learning with their peers.
Jasneet: Right. Uh, and then also the other thing around the team and doing a lot of training, right. Uh, they call it train, we call it train the trainer. So you need to ensure as I'm good with mentioning, uh, you know, [00:37:00] that person's important who's talking day in, day out. To be trained that person very well. It becomes an internal subject matter expert for the organization and that person can cross pollinate and cascade that knowledge in the organization.
Jasneet: So I think those, those are the tactics which should be not overlooked, if you will, and should also be given equal importance for any AI initiative which can have profound, deep impact. on an organization. So it is a strategic initiative. One should look at that initiative holistically, uh, and, and, you know, and then go about it.
Jasneet: Yeah,
Mehmet: absolutely. I agree with you, Jasneet. And, you know, I've again, uh, especially now because you mentioned about, uh, AI initiatives and of course what you do guys help with these AI initiatives because it's leveraging and harnessing, you know, the power of what AI machine learning can do to streamline The business operations, safe course, and all this.
Mehmet: So it's important, you know, first, you know, in my [00:38:00] opinion, to your point, the communication. So first we need to understand on a company level, why we are doing all this, right? So, so like having the end in mind, I like to call it this way. I'm a big believer in this concept. Second, and I agree very much with you.
Mehmet: If, you know, the owner or, you know, we call him the champion communicating with others, you know, on the progress. So people will start to say, because of course there will be friction down the road. People will say no. And to Ankur's point, like maybe the IT department will, will do some friction here. You know, maybe, I don't know, some other departments, the supply chain department, they say, Hey, we're busy.
Mehmet: We cannot help. So when these communications start to happen and, you know, Show the value that why we're doing this and how things are progressing down the road. I believe this would be a collaborative success for the organization implementing, you know, AI, and especially in the domain you are in. So absolutely.
Mehmet: So, uh, gentlemen, like really, I enjoyed the conversation. Like we covered a lot and this time I loved having two guests, someone speaking Ankuring, speaking about [00:39:00] the technology, Justin also speaking about the business aspect, you know, like this was fantastic conversation. And I could just remind us where we can find, uh, you know, the website.
Mehmet: Of course, again, I put in the show note, if you can just tell it to us. It's true. Gradient. ai is the website name. Yeah.
Mehmet: And of course I encourage the audience to to, um, you know, go to the website, reach out to you or to just net both of you. So I wish you all the luck and success. You know, I'm, I'm big fan and, uh, this is why we have you guys for the second time. I know you had great progress in the past year, so thank you very much for sharing.
Mehmet: Just let also your insights on the AI institutes are very, um, I would say insightful. You know, not only again for for the supply chain I think it can be applied for other verticals also as well and other cases And Ankur also what you mentioned about the techniques I think it can be applied to many other domains, but you guys will [00:40:00] focus on what you do And as I said, i'm pretty sure you're gonna do fantastic Uh in the near future and for the audience.
Mehmet: Thank you very much for tuning in If you just discovered this podcast by chance, thank you for passing by if you did You We are available on all podcasting platforms. So subscribe to get the latest and share it with your friends and colleagues. And if you are one of the followers that keep coming, thank you for being loyal and thank you for supporting the show and keep sending me your suggestions, questions.
Mehmet: I'd like to read them all. And if you are interested to be on the podcast, you know how to find me. You just message me on LinkedIn and I will try to get back to you as soon as possible. Thank you very much for tuning in. We'll meet again very soon. Thank you.
Jasneet: Thank you so much. Thank you. Bye bye.
[00:41:00]