#434 Managing AI Risks: Jim Olsen on Governance, Compliance, and Business Strategy
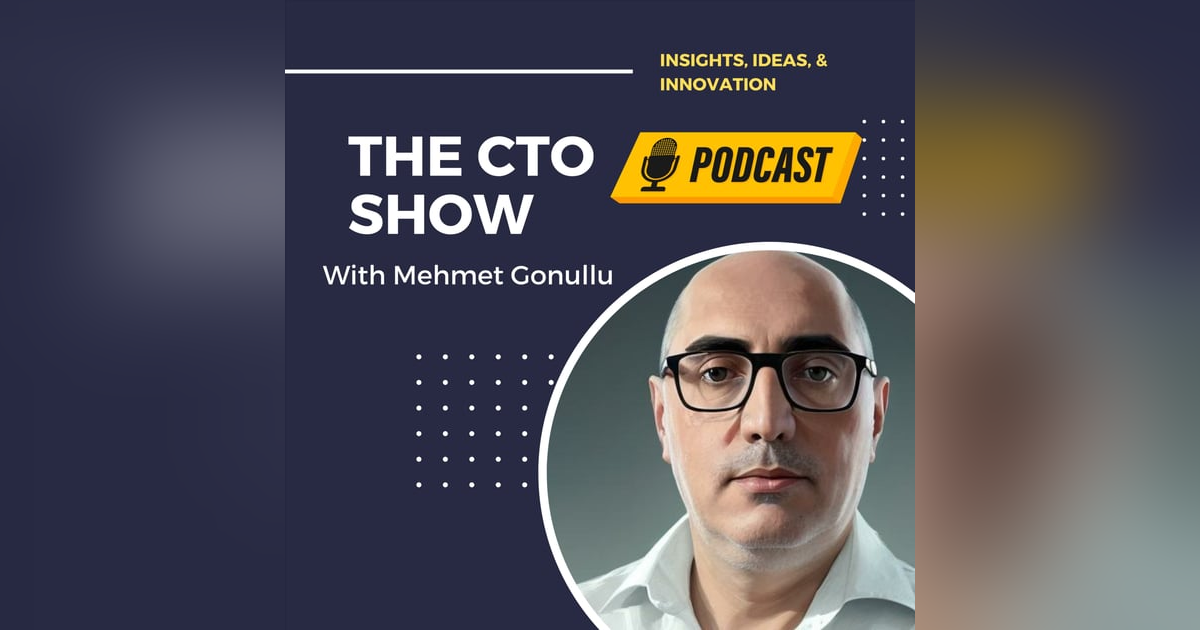
“Most companies can’t answer the fundamental questions about their AI models—where they’re used, how they’re performing, and if they’re compliant. That’s where AI governance comes in.” — Jim Olsen
In this episode of The CTO Show with Mehmet, we dive deep into AI governance, compliance, and risk management with Jim Olsen, the CTO of ModelOp, a leading AI governance firm. As AI adoption accelerates, enterprises must navigate the complexities of AI governance, regulatory compliance, and business strategy.
Jim shares his journey in AI, the importance of AI governance, and how enterprises can balance innovation while mitigating risks. Whether you’re a tech leader, founder, or executive, this episode provides valuable insights into securing AI models and ensuring responsible AI use in businesses.
Key Takeaways:
✔️ AI governance is not just about regulation—it ensures AI models drive business value.
✔️ Most enterprises lack visibility into how AI is being used internally, leading to compliance risks.
✔️ AI models are non-deterministic, requiring monitoring and lifecycle management.
✔️ Regulatory frameworks are evolving, and businesses must be prepared for compliance.
✔️ Balancing AI innovation with risk management is crucial for long-term success.
What You’ll Learn in This Episode:
🚀 Why AI governance is a critical priority for enterprises.
📊 How organizations can track AI models and ensure compliance.
⚖️ The evolving landscape of AI regulations in the US and Europe.
🛡️ Strategies to mitigate AI risks and protect business reputations.
💡 The role of AI in business decision-making and automation.
About the Guest – Jim Olsen
Jim leads the technical innovation and design of the ModelOp Center platform. He
also is integral to advising ModelOp customer CIOs and CTOs on requirements to
better support their IT operations as they execute on digital business strategies that
often strain technology infrastructure.
Prior to ModelOp, he was Director of Software Development at Think Big, a
Teradata Company for the Americas consulting organization, and responsible for
the design of their Analytics Ops framework. Jim has also held technical design and
architect positions at Qualtrics, W.J. Bradley Company, and Convasant and was a
Distinguished Engineer at Novell.
Jim holds a Bachelor of Science in Computer Science and Psychology from Clarkson
University and currently has two patents in his name. Connect with Jim on LinkedIn
at
https://www.linkedin.com/in/jimolsen/
Episode Highlights & Timestamps:
⏳ [00:01:00] – Jim Olsen’s background and how he got into AI governance.
⏳ [00:03:30] – Why enterprises struggle with AI oversight and compliance.
⏳ [00:06:00] – The disconnect between AI adoption and C-suite understanding.
⏳ [00:09:00] – AI regulations: What’s changing and how businesses should prepare.
⏳ [00:14:30] – Risks of deploying AI models without governance.
⏳ [00:18:00] – Generative AI: Potential, pitfalls, and business impact.
⏳ [00:23:00] – Best practices for AI governance in enterprises.
⏳ [00:28:00] – The CTO’s role in balancing AI adoption with compliance.
⏳ [00:35:00] – Future of AI: Agentic AI, automation, and evolving risks.
[00:00:00]
Mehmet: Hello and welcome back to a new episode of the CTO show with Mehmet today. I'm very pleased joining me Jim Olsen Who's the CTO of ModelOP a leading AI governance firm for enterprises? Uh, Jim, [00:01:00] thank you very much for being with me here today on the show The way I love to do it is I keep it to my guests to a little bit, you know Tell us more about them about their journey And what they're currently up to so the floor is yours Jim
Jim: Okay.
Jim: Absolutely. Yeah. I'll kind of intro a little bit up front about how we, uh, how I kind of came to a ModelOP and the whole idea of AI governance. Um, and you know, it's, it's been quite a journey. Uh, first job programs was 13. I've taught myself at nine. And as you can tell, it's been quite a few years since then.
Jim: And, uh, I've seen the full gamut of everything. You know, I started with the old nine track tapes and recorders and, uh, Thermal printing paper type terminals and all of that all the way up today where we're seeing AI and we're seeing, you know, Billions of parameters inside these models and that well, that whole journey.
Jim: What's interesting from my standpoint is, you know, I worked as a distinguished engineer at Novell for quite a few years and [00:02:00] worked actually designing protocols and implementing things at the lowest of levels all the way up to the first versions of Java and working with, uh, People like Bill Joy, et cetera, directly, um, around those concepts.
Jim: Uh, so very interesting. And it was very much about, uh, programmatic and protocols and low level things. Uh, but what I've seen specifically is, uh, my previous company that I worked for, we were working with big data. Uh, you know, that was really became an enabler. I mean, there was a lot of talk around building data lakes and all of these things and that, but the real value wasn't in just hoarding data.
Jim: It was. Now we have all this data, we can start doing interesting things. So I began to look at that and how some of our customers at that time were using data to train models, et cetera. I realized we were in the infancy of basically data science and models, much like think back to the old days in software engineering, where we would actually go out and kind of just code up something on our [00:03:00] local computer and just push it out to production.
Jim: No thoughts, you know, no reproducibility, no CICD pipelines, no, those kinds of things. What we've seen is in the data science world and all these other things is Yeah. Now, in fact, we're at that point where I was working with people who had data lakes, who scientists were just pushing models straight out to production into customer sites, et cetera, with no idea of where these models are, or that it's naturally organically being used in the business to accomplish tasks without any knowledge, oversight or understanding if that person leaves or, you know, whatever.
Jim: That knowledge is lost those those how did that model come to be and now it made a business decision And if that were to come under scrutiny How do I actually go out and prove that some due diligence was done? And what went into this model and that I didn't use inappropriate data. I didn't whatever So that really led up to this idea that we needed something in place uh to actually provide the Unique [00:04:00] insights into models rather than just programs where they're deterministic in nature.
Jim: A lot of these newer AI models are non deterministic in nature. So how do we track them? How do we know where they're being used? Vendor models come into play because most people aren't running chat GPT for locally requires a lot of resources. So how do I track those and understand where they're being used?
Jim: So that's where the whole idea of AI governance. And we, we. Tend to think of it in the news as a punitive thing where it's like the government's coming down on you. But no, it's also internal understanding where my models are, where they're things, are they performing for my business? Who's using them? Are they generating, are they generating revenue for me basically, as opposed to costing me a ton to run them, et cetera.
Jim: And we find most companies can't answer those questions. And, you know, that's kind of gave the birth to the idea for model ops. Being the solution, the first kind of pure play AI governance solution that's out there that, you know, gardeners recognize that we're kind of the big pure play one that can holistically do that within your enterprise.
Jim: And [00:05:00] that's where the ideas came from. That's the journey that kind of led to this. And I feel it's like the next forefront in kind of the inside of the DevOps world, which is all around programs. This is around how do you manage your models as business assets?
Mehmet: Right. Now, that's a very fascinating, you know, intro and, you know, how things came to here.
Mehmet: Um, another thing, which is kind of setting also the stage for the audience and, you know, because you mentioned about, you know, how we started to collect the data, we put them in the data lakes and, you know, we start to try to figure out what we can do with that. Um, There is something also about the transparency and you know, like Jim, like Accenture CEO mentioned that only less than 2 percent of the C suite understands how AI is used in their organization.
Mehmet: So in your opinion, why there's such disconnect? Like, of course, you know, um, I can understand that sometimes. You know, something comes from the board, [00:06:00] right? Hey guys, like we, we, we need to rush out. Like we don't want to be like left behind and we need to adopt, you know, AI and whatever. So this is, this is like one, one side of the story, but from, from, from your perspective, why do you think there's a disconnect?
Mehmet: And you know, how organization can. Take the bridge, uh, you know, to take, you know, actions to bridge that gap.
Jim: Yeah. Well, a lot of it comes down to, uh, there, there's a bit of, yeah, there's the, the, the CXOs who, uh, want to ride the AI hype wagon and jump on and do something. But, you know, most people don't really fully understand what AI can deliver, what it can do, its limitations, how you measure it, all of these, uh, other kinds of concepts.
Jim: So those efforts that push down from don't really come with instructions. So what you're finding instead is I refer to it as water finding its own level. Um, basically you're finding natural organic usages of AI where people want to take [00:07:00] advantage of what it delivers. For instance, one of the big ones I see is creating summarizations.
Jim: Uh, it's a very natural organic case for LMS. I've got this huge document that got dropped in my deck, uh, desk, and I just want to, uh, understand some of the key points that I'm busy. Yeah, drop it in there. Plug it. Let it summarize. It gives me a few key points to see if it's relevant. And then I can dig in deeper where it makes sense on that.
Jim: So we're seeing, you know, these natural organic uses. And of course, within coding, we're seeing a lot of people wanting to use AI for coding, which comes with both negatives and positives. But again, they're not understood. So where the disconnect comes is this organic usage throughout the organization of water finding its own level means This isn't documented anywhere.
Jim: We, we don't know. I mean, for instance, uh, I've heard of, uh, one hospital where they discovered patient, uh, data was being uploaded in the chat GPT 4, which is a kind of a big, uh, no no, um, to generate, uh, the summaries for that. And they found that out and had to block the domain to stop this from happening.
Jim: So people are [00:08:00] taking advantage of it as a time saver, but You can't just open the open the gates and let everybody do anything because there are real privacy concerns, et cetera. So that's one of the reasons our product we what we call minimal viable governance. The first very first step is understand what models are being used within your organization and get a basic inventory by use case.
Jim: Not by model, you know, not chat GPD four. Yeah, that's part of it. But what uses are these being used? So, you know, everything starts with the use case before you even start using AI and understanding how they're being used. And that visibility is just not readily available to most CXOs because if you're lucky, maybe somebody has a spreadsheet somewhere is what we typically see.
Jim: And that's where our product comes in and kind of fills that gap, provides a comprehensive detailed inventory of usages and what it's. Reviews have gone on etc because you need that knowledge.
Mehmet: Great. So jim, you mentioned an example, which you know is relevant to the next question Like I I was going to ask you you [00:09:00] mentioned hospitals, right?
Mehmet: So so when we talk about any place like healthcare banking systems government entities, so we talk about regulations also as well, right so You know, what do you think the AI regulation so there was a lot of debates and I know and maybe I'm sure like you you're exposed not only to the u. s regulations.
Mehmet: So also like, you know, Europe everything Like europe, it's another story and some people they say yeah, they are putting a lot of regulations that making, you know, people's lives hard. I don't know. Right. And there's a lot of talk and even these AI companies that they are producing the LLM models, we see them always, you know, saying, Hey guys, like we want also to be part of regulating this.
Mehmet: And we want to be part of, you know, this community that works hands in hands with government entities, with experts in the domain. So, [00:10:00] With all the hype that's happened in the past two years, and I think, you know, now we are reaching a phase where these LLMs are going to the next step. I don't want to call it, you know, AGI or ASI or whatever, so maybe we can discuss it in another question.
Mehmet: From your perspective, If we talk about regulation, so what, where are you seeing things going? And are enterprises, you know, preparing well for these changes today?
Jim: I'll give the, the, the answer quickly is no, uh, organizations are not prepared for this and, and understanding it partly because of the regulations themselves.
Jim: You know, we're not seeing the EU act. Is a fairly unified, um, basically application of regulations across the body. But what we're seeing in the U S for example, is fragmented rules kind of all over the place. So for instance, the, you know, Texas has a specific healthcare act, uh, talking about, uh, usage of AI [00:11:00] or machine, machine learning models in healthcare and that they have to be documented, et cetera.
Jim: And then other States have different Colorado has their, uh, acts as well. And they all have different nuances to them. Uh, you know, the fed. federal act in the U. S. Is more targeted at foundational models. Um, you know, the foundational models that that's more container containerizable and gets more. I think it gets into more arguments about what kinds of regulations should be in place there because it's not as quantifiable.
Jim: They can be used for tons of different things and for different use cases. Freeform spitting out, whatever, maybe an acceptable use case, you know, maybe a creative act or maybe that kind of things. But what we're seeing where businesses utilizing these foundational models and any machine learning model, in fact, are not prepared for is now, if I want to take those, uh, foundational models or even a trained machine, traditional trained machine learning model and use it to make decisions about an individual's healthcare, for [00:12:00] instance.
Jim: Maybe that does deserve a little bit more scrutiny, um, than just the foundational model doing things on its own. Uh, because, uh, we, you know, it affects people's lives. Uh, you know, for instance, uh, yeah, I can't remember the county, but somewhere in Nevada, they're talking about Uh, basically, uh, basically approving or denying unemployment insurance, uh, primarily using a machine learning model that that hits somebody in the bottom line.
Jim: If there's not a recourse or a person in the loop to review this because it becomes kind of this automated justice for lack of a better word. And I don't think any of us want to fall under that. Maybe they can help make these decisions. But you know, that's what a lot of these regulations are Putting in place that, you know, automating, because it gets even a little scarier when we get into a gentic AI, and it's actually just going to go out and take the actions all on its own and interact with different systems.
Jim: And if there's not transparency in how this is happening. [00:13:00] You know, it could, it could just decide, well, uh, obviously the best thing is to deny everybody, uh, unemployment insurance because that saves us money, you know, things like that. So you do want to make sure we understand where these things are being used, but tied back to the use case, because another use case might be an internal thing that only affects internal employees, and maybe it can be a little looser than that.
Jim: If I've got another thing that's, uh, using the same model, but it's going out, it could affect my brand. Know, put out bad things and, and statements. Uh, uh, you know, there's been a bunch of famous examples of, uh, like the, uh, McDonald's when they launched their AI initiative at their food kiosks. You know, it, somebody who came up to order it, just kept adding orders of fries and, or chicken nuggets, I can't remember which, but just kept adding more and more to the order and the case, no stop.
Jim: They would add more to the order. You know, this stuff hurts your brands and, uh, you know, can really affect your business even if it's not drastically affecting somebody's life. cost your company money. So having both an understanding of what you're using [00:14:00] models for and then understanding what the regulations say about that and having that ability to tie those together and identify those risks is critical.
Jim: That's what our solution provides is that ability to do that in an automated fashion and ensure that those risks are addressed. So that way We help companies to be prepared for these acts as they continue to come on, because they will continue to come on, especially when we see them making life or death decisions literally for customers.
Jim: I think all of us can understand where the regulations may apply there, as opposed to foundational models. And it shows me a dirty picture or something that's not nearly as big of a deal of as denying some of the health care,
Mehmet: right? Again, within the regulation, but this time, because for some of the folks who might think, okay, listen, I'm not using anyone else's LLMs.
Mehmet: I have deployed, you know, my local LLMs. Okay. Maybe they are rich. They have the infrastructure to run. But there [00:15:00] are still some risks there, Jim, right? So, yeah. So let's talk about these risks. Like what can go wrong and how, of course, from model of perspective, you can help them.
Jim: Yeah. Well, I mean, to be honest, I think we're actually going to, I'm already seeing customers deploying, uh, like llama.
Jim: versions, et cetera, locally. Um, and, uh, there's a lot of growth in what I call the medium, large language models, uh, where they are getting, uh, small enough. And, uh, we recently seen NVIDIA and it's digits project, uh, project where it's a 3, 000 box that can run, uh, a very large, uh, very. Reasonable sized L. O. M.
Jim: That can perform many of these tasks and the performance of those medium models is actually getting quite good. So I think you will see more and more people running these, uh, these assets locally where they're concerned about pushing their data off into the cloud, or it's just not even allowed, like in the case of health care, etcetera.
Jim: So we will see this usage. The danger in most of these is, uh, you know, there's no [00:16:00] explain ability. Um, I like, I like to refer to LLMs as fluent, but not factual. Um, they don't particularly know exactly what they're saying. They're not reasoning when they do these things. It's a mathematical model that basically predicts, uh, what makes sense to say based on what.
Jim: Was asked. Um, so with that, we do see hallucinations. We see making up facts. We see that that will improve somewhat, but it will never go away. There was a very interesting article I was reading where recently we're seeing now that some of the, uh, GPT. I think it's the one model will actually do its intermediate reasoning in Chinese because it's got so much training in that and that Chinese language actually tokenizes better.
Jim: So you'll see it switch to Chinese and then kind of switch back to English. Or if you do search some Unicode, sometimes it will always respond in Chinese. So there's very odd behaviors and there's no explainability. So it's Difficult to establish [00:17:00] trust with both the end user in the company around these models.
Jim: So you do have to have a process in place that basically analyzes these models, monitors these models. Uh, you know, it gets them reviewed, ensures reviews occurs, identifies all these risks, etcetera, like our product does, and basically tracks that There's enough there. Somebody's really thought about this from the use case perspective in order to build that trust with the user and have the abilities and things.
Jim: Some of those real time guards like guardrails and all these other things that are in place certainly. But again, will people trust using your product if you don't put the effort in to show you did the most due diligence you can? And that's really where we're seeing that around
Mehmet: Super, super informative, I would say, uh, Jim.
Mehmet: Now, Back to, you know, the generative AI, you know, potentials and pitfalls perspective. So [00:18:00] some, some organizations are seeing it as a way, you know, to drive, you know, more digitization and, you know, the digital transformation while some they think, no, let's, let's wait a little bit. Um, some of them, and I heard it by the way, from, from some executive, they said, Um, actually, we we don't know if we adapted like how we're going to scale it, right?
Mehmet: So we might start with small use cases, but how we can take it to the next level. And this is maybe it touches on the part which you just mentioned now about, you know, monitoring how they can see if they are getting the benefit out of it. So if we want to put this, you know, translate the technique technology part into like the business outcomes.
Mehmet: And you mentioned in your introduction about, you know, if they are getting the ROI, is it increasing their revenue? So what are the pitfalls you've seen? And, but, you know, if you can tell us, what are the potentials that you think like [00:19:00] still can be uncovered in utilizing generative AI?
Jim: Yeah, absolutely. I mean, generative AI has great use cases.
Jim: Um, I mean, I've seen several like there's immediate natural fits. A great example of this is one of our financial clients. Uh, you get a big prospectus in on a new fund or a commodity or a report on a commodity or something. Uh, you know, minutes cost you money. So being able to summarize a large, large prospectus or something into the salient points in a report.
Jim: In seconds, uh, is literally can make or make you a bunch of money on that kind of things. If you can do it more accurately than your competitors, there's a great example of where there's a natural fit. I've also seen the other side where, yeah, as you mentioned earlier in one of your questions where you get the, the C suite just demanding, we want to be on the AI.
Jim: Bandwagon and want to do something there. Come up with something. You know, that's the wrong [00:20:00] approach to any development solution. Never mind, uh, generative AI and specific, but even the software, we all know how those projects turn out. They go on forever. Instead, again, I keep Going back to this. But you start with the use case of the problem you want to solve.
Jim: You don't even identify that's an A. I. Use case yet. You may flag it as potentially an A. I. Use case, but perhaps a traditional machine learning model makes more sense or even a simple decision table. I mean, those are still valid and very useful for certain use cases. Then you analyze the use case and say what technology makes sense and how much is it going to cost me?
Jim: And you do need to understand, like, yeah. Okay, what's the cost per request? What's the profit per request? What's the saving? Um, I mean, a big area where we see, uh, when you take this kind of approach is helping, um, for instance, customer support representatives get to information quickly, uh, using a rag architecture into previous support cases.
Jim: Or support documents, et cetera, where they can type in the customer [00:21:00] problem is natural English language and get some pointers, uh, and maybe links to the specific documents by using the vector database and actually referencing where it got that information from natural fit makes your, it, what they've found is it makes kind of their middle performers, high performers, because the high performers put the information in the first place.
Jim: Now they can leverage that and pull that in. So that's where we can see an ROI of having to, you know, not. Try to find every superstar I can get, you know, make every one of my, uh, customer support representatives more engaged and more powerful and, and leverage within the industry. So again, it's understanding the use case, is AI applicable to, uh, doing that use case and how much is it going to cost me to run this AI, um, and, and investments in there.
Jim: But. As I said, especially these advances in the medium language models and being good enough, especially in a rag solution, I think that cost is going to continue to come down and you're not necessarily going to have to have the latest, greatest model to still, [00:22:00] uh, achieve that revenue, but you got to understand that you don't understand it, you're never going to get there.
Jim: So again, that's why you got to have that inventory and what the costs are and the use cases, et cetera, to make those determinations.
Mehmet: Right. So if I want to go back a little bit to the governance part, right? So, um, for organizations that they might just started, right? So is there like, because you know, in the cyber security world, there's a lot of frameworks that people utilize, like the NIST framework, you know, there are like also some best practices, I don't know, like ISOs and so on and so forth.
Mehmet: So in the AI world, what, what that looks like?
Jim: Uh, it's tough right now because everything's kind of emerging, Sarah. And then that's one of the reasons we developed this solution is because an individual trying to keep up with all the regulations popping up left and right, state level, international level, federal level, et cetera, is challenging.
Jim: And then also understand how [00:23:00] they're applicable to their individual product. So that's one of the reasons, you know, we have this product, and then there's, there's a few others out there, but not very many, um, and we have this ability to basically provide kind of checks and balances, questionnaires, et cetera, that a developer can answer, uh, starting when they actually just start even developing that use case, and we can then identify potential risks where they happen and, and how you mitigate them and create those actions and manage that.
Jim: But then further continue to follow the model through the full life cycle, um, out to deployment. And cause there's, uh, even things that, oh, there's an annual review required when you get into financial, uh, situations is regulations apply that. We love to talk about the, the AI regulations, which are out there, but.
Jim: You look at the financial industry. They've been under Frank Dodd Act and all these other things for a long time, heavily regulated for the use of their machine learning models in the traditional sense. Of course, AI falls under that as well, where they've had to comply with these and these large [00:24:00] institutions had to build their own massive systems themselves to manage this process.
Jim: That's not practical outside of being one of the top Financial institutions. So that's why products like ours that exist that can help you do that are just vital, um, to being compliant with those regulations. I mean, fines can get huge. I mean, uh, there was a bank that just got fined. Billion dollars for not following the regulations.
Jim: Then I imagine we'll see more and more of that in the health care, although they seem to be aware of it. They just don't know quite what to do yet. And that's why our product helps them be compliant. So they don't have to worry about that kind of thing.
Mehmet: Right. So, so, and this is, I think, and correct me, Jim, if I'm wrong, this would help them to create kind of the balance between, um, the need to innovate, right.
Mehmet: And that, you know, at the same time, not breaking the rules. So, and because like every time we have a new technology in place, there are always this debate, um, [00:25:00] okay. Like don't, don't innovate too fast. So we don't want to. You know, get a penalty or a fine from, from, from some place. Uh, but you see, you hear the other, you know, I would say side of the room, they will be saying, no, we need to innovate fast.
Mehmet: Like we need to get this out. So like, is there any. kind of best practice also to do this balance between innovation and making sure that we are not breaking these regulations?
Jim: Yeah, well, that's where it comes down to the use case. We can certainly innovate in areas, um, and nobody wants to stifle that.
Jim: Most people don't want to stifle that as far as making better models that can do more and are more interesting, more engaged, uh, you know, more engaging, um, et cetera. But where it comes down to is, you know, we want to be careful in the areas where it can really affect people's lives. Um, like there was, was a model deployed in the healthcare industry that was discriminating against black [00:26:00] individuals for long for kind of getting more emergency.
Jim: Care quicker for whatever reason the bias got built into the model. Um, and it was shown the model was thrown out because of that. But it was being used by many, many large health care providers. These are the kinds of things where I think we can all agree where regulations are good to make sure those kinds of things don't happen.
Jim: So yes, it's a tricky balance in that you don't want to destroy productivity or even things like the opposite where it's predicting cancer more accurately in, in scans of cells than humans are, uh, when they're doing it. Uh, so, you know, how do you, we don't want to stop that kind of thing because that's helping save lives, um, on that kind of thing.
Jim: So how do you create that balance between the two? And it's a, it's a tricky tightrope and I don't think there's a magic answer. That that anybody can come up because naturally any regulation is going to slow down the process because now I have to prove we're compliant. But that's where tools like ours that helps automate that process and reduce the time it takes [00:27:00] to be compliant with those regulations and only hit the things that.
Jim: Are likely to have to come under this kind of scrutiny while other areas where you're using it that don't have those kinds of implications. You let that run more free and let that run faster. But you know, you need solutions like ours to basically help automate and shorten that. So you don't have this backlog on some poor guy's desk of a million models.
Jim: They have to review to see if they fall under the regulation. Uh, automating that alone helps reduce that time window and the burden of regulations and hopefully allows for innovation.
Mehmet: Right. So, Jim, uh, how this makes the life of a CTO easier or harder? Because, you know, CTOs, as usual, and you're a CTO yourself, so You sit on, on, on, you know, this two sides of the table also as well, where, you know, you have to put, you know, the technology vision, you know, and so on, [00:28:00] but at the same time, you know, you, you, you manage, you have to also take care of the business side of it.
Mehmet: So with, with, with these. Two, you know, um, double edged, I would say, roles that the ICTO plays. How have you seen, you know, this affecting also their day to day decisions, maybe, or I don't know, like the initiative that as ICTO they need to take?
Jim: Yeah. Well, as you point out perfectly, the kind of crazy balance of the CTO is, uh, yeah, you need to be leading bleeding edge of technology, but then also balance that back to, is this the right thing to do for our company from a tech, from a business standpoint, a profitability standpoint, even, uh, as opposed to just, wow, that's cool.
Jim: I mean, there's that side of us. I think all of us, Both CTOs who came from a hands on background and continue by myself, I'm still hands on, I still write code, et cetera. It's like, yeah, the, the, the shiny toy you want to play with that was really cool and promising, et cetera. And we all love that, but you're [00:29:00] like, yeah, but it's going to be really hard to hire engineers in that language because so few do it, it's not the right thing to do for our business.
Jim: So that, you know, it's a simple example. We can all understand, uh, about how you, you. You have to balance that. Those two factors. Additionally, that that's true with AI usage, et cetera, as well. A lot is coming on to CTO that is desks. There's stuff being announced every day. That's new. I mean, obviously, the most recent hype is around agentic AI and all that, you know, chat GPT announced today that they're allowing automated schedules of tasks to go out and and and Um, Uh, remind me of things later or look up tickets.
Jim: I haven't yet played with it to know the depth that goes to, but there's, these are the examples of everything. Salesforce is talking about their agentic AI and what they're offering their next hour and what impact does this have on the business? So that's where, you know, you really do need the tools to understand.
Jim: where A. I. Is being used or has potential to be used within your company. And that's [00:30:00] why our product isn't just about A. I. We were doing, uh, basically matricial machine learning models and even excel spreadsheets that make predictions. We have the ability to inventory those and do that and have been working with financial organization with those for years before that.
Jim: But you know, we saw a coming built that in early and etcetera as well. And it's another thing that's in there. So the CTO needs to have that that Vision into where it's being used, where it could potentially be used. It makes sense because there's a lot of areas where maybe it's not being used and should.
Jim: Um, to save the company money from the things that's the job of the CTOs to identify technologies that help the business and then help make it a successful business for a variety of different reasons. Uh, you know, I think there's a lot of pressure on CTOs to just use AI because we want to say we use AI, but, you know, that's the job of the CTO to also push back and be like, yeah, but for what?
Jim: What makes sense for us? Because we could throw a lot of money down this hole and it didn't make sense [00:31:00] and this actually hurt our image in the long run. So I think CTOs are facing that challenging balance of the pressure from above to say, we use AI, pressure from below, we want to use AI. Okay. How can I marry these up and identify the right places where we absolutely, from a business and a technology perspective, use AI in the business to make the business better.
Mehmet: Right. Yeah, it's you have a very tough, tough job, Jim, and all the CTOs as well, because you need to keep this balance. Now, you know, I wanted to ask you like a couple of minutes back and now it's the time. And you just mentioned, you know, about how things are moving very, very fast, right? So we started it.
Mehmet: And actually people they say, of course, like AI has been with us for since the 1950s. But I mean, the acceleration that we saw, especially after the, uh, transformer paper came [00:32:00] out and then how open AI and all this, and, you know, we expected and everyone expected that, yeah, like we have a hype here, but then now we are starting to see things accelerating very, very fast.
Mehmet: And from, Someone like you, Jim, who's experienced and you've seen it all, as you said, uh, where are we heading with, with, uh, with AI technology? So, and I'm asking you from a technology perspective and also like in general, I mean, from non businesses, because now, you know, OpenAI keeps, you know, talking about, you know, how they are close to AGI.
Mehmet: Um, Sam Altman talks about the one person company. The other day we saw Mark Zuckerberg talking about, you know, the mid level engineer. And of course they are mentioning agentic AI, but I think there's more to come. [00:33:00] So what are, you know, your Overall thoughts about, you know, this acceleration and I don't call it prediction because honestly, no one knows.
Mehmet: Right. But I mean, like we can, we can say we think like it's going to go this way. So what are you seeing in this space, Jim?
Jim: Yeah, well, I mean, as you mentioned, neural networks have been around for a very long time. I think I programmed my first one in the 80s to recognize handwriting or something like that.
Jim: But what the big evolution was, as I mentioned earlier, is the availability of the data to train them on. Um, before it was very difficult to collect enough diverse data, um, to do anything mean meaningful with, uh, neural network trainings. You know, we could have specific data sets for very, uh, very purpose oriented things or run traditional logistic regressions on them or those kinds of things, but they were limited.
Jim: data sets. Now we have massive data sets of language in many different languages readily available at our fingertips just on the World Wide Web. Never mind in like, uh, just think it's like the Gutenberg [00:34:00] collection of books. I mean, every how many Words are there available to train things on, and now we have the bandwidth and the data to move them to do these trainings.
Jim: So that's what's really kind of, I believe, lit the fire under that is labeled data, because unlabeled data is not very useful either, but we have self labeling data. We hire companies to, to. Just sit there and label data all day and et cetera. So there's this vast training data set that's been out there, and that now is continuing to evolve.
Jim: And now that people are interacting with these LLMs, they're basically creating their own labeled data sets that they're using to retrain their own models. So you've created a kind of feedback loop in here. But what we're starting to see is, um, and, you know, again, There can be dis, any, there can be disruptive changes in what's going on now, but it does seem we're, we're starting to plateau on just throwing more data at it.
Jim: Um, we're not seeing the LLMs improving vastly by just, Oh, just give it more data. Uh, we [00:35:00] are seeing a little bit of plateau, but now I think we're going to start seeing is for the ability and I've been a big proponent of this a long time ago. It is an interesting topic. Um, you know, there's both. Not as in positives, you know, uh, back in the nineties, I was working with Bill joy and some stuff, and he was talking about genie and doing Java agents and having agents that could communicate and discover each other and, and inter operate, did some early work on that.
Jim: And this idea of basically things being able to talk and figure each other out without having an established API, like we've always had in the past is very interesting. That's going to open up a lot of possibilities. And that's that's the premise behind agentic AI is, well, I'll figure out how to talk to you and get the information I need to then do something and then auto generate a form based on my guess.
Jim: Now, obviously, that that's very interesting from from a, um. Mentality perspective and just thinking about the problem. It's like, oh, that's kind of cool. I don't really need an API. I [00:36:00] don't really need to figure this out. I'll just let that work it out because it's it's it's form of its own language. You know, web form is something I can understand and do so.
Jim: I can just figure this out and get the information I need and they can't really stop me. But what's a little More concerning to me is the non deterministic nature of that, that kind of scares me a little bit of having been a programmer for years and years and years of, okay, is it doing the right thing and how do I determine it's doing the right thing?
Jim: Let's say I've got a simple thing, all agentic AI works on this idea of a chain of events, you know, one thing figures out one piece and passes it down, next piece figures out the next piece, let's say it's 98 percent accurate. That means 2 percent error change. Now change that down. The next one's 98 percent accurate.
Jim: Well, you get the idea. I keep losing 2 percent every time I go down the chain. So how do I manage that? And I think, you know, for that technology to really take off, I think we're going to have to see a lot of advancements in understanding accuracy of these [00:37:00] responses. And that will also feed back into the other example, which you mentioned is making coders better.
Jim: I've, I've used a lot of these. I'm a. You know, not toot my own horn too much, but I'm a really good programmer. Uh, and basically The tools are helpful in some ways, but I also can find that they can slow down your best programmers. They can help move your middle programmers up to a degree. They make your lowest programmers absolutely the worst.
Jim: They become like stack overflow cut pace programmers. Yeah, it's like they don't, they just take the answer and don't review it. So they just go, oh, yeah, that looks good. And don't understand it. So we do have a danger there of making sure that yes, these tools are tools that help a programmer, but they need to go back and review and understand what it is and what it's really doing.
Jim: So there's, you know, cost benefit will change. I'm sure they will continue to improve. And I've seen that just over my career with some of the, just the simplest of auto complete things, but [00:38:00] half the. You know, depending on what I'm doing, if I'm doing some novel piece of code, the other is getting in my way.
Jim: And when I ask for answers from, uh, you know, something as simple as, uh, calculating, uh, uh, uh, uh, basically like a compounding interest or something in, in Java, I've tested it. They'll come back to me using doubles, but you can't do, you need to use a big decimal because otherwise you, you have floating point, point arithmetic problems.
Jim: So they'll continue to improve and continue to do that. Um, but I always believe you'll still need it. A human in the loop, and that's where I get to the genetic AI until we can solve this error problem. It seems like, yeah, I wanted to go out and find all this stuff and then maybe come back to me the consolidated list that I can then kind of go.
Jim: Oh, that looks reasonable. And I think, you know, Processes that enable that and continue to do that are going to then help the technology really take off and building trust. And that's where our product by understanding all the pieces of the components, what's going on there, having those in the inventory, et cetera, and that [00:39:00] uh, Yeah, it can help you establish trust in that these individual pieces are performing correctly and are there so you can have some confidence.
Jim: If you don't have confidence in the solution, people aren't going to use it.
Mehmet: Absolutely. And I think, you know, to your point, I'm just going to give an example. Uh, which echoes what you said, uh, Jim. So actually I saw also like someone I was asking on LinkedIn the other day. So my, of course, like I'm not an expert, but what I've seen to your point, it seems that these models, they start kind of a degradation, you know, after a while.
Mehmet: Um, and they become, I call it lazy, right? So, and the example that I, I tried myself to see, I just gave a screenshot of a Wordle game. You know, the, you have to guess the words. And even, although like, it's very obvious that I know the AI can. [00:40:00] You know, can understand, you know, what, what, what is that, uh, still, for example, I'm saying, look like this is a five letter words.
Mehmet: It contains an I and an L let's say, but still what I'm saying, suggest what that might be a fit. It's going and suggesting words that doesn't have these two letters or for example suggesting words that have Seven letters and I and I gave this example to someone, you know saying oh like it can be doing fantastic stuff I said we still need some time because it's it doesn't understand the context.
Mehmet: It's taking a text and trying to As to your point, like find something that fits and spit out something relevant to it. Uh, which first, which is good. Don't get me wrong, but I'm happy you mentioned Jim, you know, about this vision of how us as humans, we will be always be there. to give the, we give the feedback to, to the machine learning model, to the LLMs, whatever, [00:41:00] to, to, you know, steer it back to, to where actually it should be going.
Mehmet: So this is, you know, just my, my, my two cents source on this. Now, um, if, if I want to ask you, Jim, like about, um, for, um, you know, model op, like where Other areas, like, do you think you could, uh, tackle or touch on in the future? Uh, which are not today covered by you
Jim: Yeah, one of the the big plans we have down things is we plan to leverage ai ourselves more On that kind of things again, because especially we deal with the regulatory space We're careful about how we generate things what we generate etc.
Jim: But for instance, let's say We're in the future. Uh, it's a lot of, uh, paranoid governance out governments out there. They put tons of rules in place and you have to and you're an international company complying with, you know, potentially hundreds of regulations [00:42:00] that come down onto your desk. Obviously just generating those documents alone.
Jim: So for instance, we do have a document generation service that right now allows you to pull data into a form. But we've actually already started working on, uh, basically trainings, uh, more of a rag kind of training rather than reinforcement learning, but more getting these, uh, these into, uh, our document service where we can auto generate, uh, documentation for all the different regulations based on a common set of answers that you've provided.
Jim: So you don't have to go through and do every one. The idea of being, again, we fill it out, but then somebody. Reviews it and goes, yeah, looks good or not on those kinds of things. So that's, that's the usage of AI. I think people want to see is, is helping you to do your job better, not replacing on that kind of things.
Jim: I mean, obviously, I don't think any of us want to be replaced. Now, the reality is. Like any other new technology, let's get real, uh, jobs as software programmers for years have been about [00:43:00] automating tasks that were done by people, uh, before, and it does eliminate jobs, but those people move into additional roles, and I think we will see some of that with artificial intelligence for sure, but it will create new jobs around, yeah, now somebody's got to look at what came out.
Jim: Is this stuff reasonable, et cetera? Um, and there's other areas that are going to need to be worked on when we remove some of the kind of, uh, you know, uh, day to day individual items on that kind of thing. And that's, you know, I think that's, that's the future and where our solution is going to do is to try to help these companies do more with less.
Jim: And that, that's the reality of everything out there. But right now, the interesting thing with our company is. Nobody's doing it, so we're not replacing anyone. Uh, you know, it's like people need to be doing it, and they aren't. And, you know, it's going to come down on them. So, you know, they already don't have experts in this place who can help them do that.
Jim: So that's why we want to make our solution as much of, uh, be the expert for you. [00:44:00] Um, so you don't have to learn all of this stuff. And, you know, we're, we're, we're wedging a lot of the kind of AI solutions into. Doing those kinds of things in a flexible manner that can keep up with regulations as they continue to come out So that that's a big area for us where we ourselves are going to be leveraging ai more
Mehmet: great uh We are coming to an end james.
Mehmet: So As usual, I ask all my guests so any final words you want to share with the audience and where they can get in touch
Jim: Yeah, absolutely. Uh, you know as I said, uh This is a rapidly evolving space. It's kind of an exciting time from understanding, you know, that this kind of power is being put into everyday persons.
Jim: Tans, you can go play with the A. I. Yourself. It's not some big scary thing can click the little copilot button if you're in windows or you can play with chat before through the web interface and get an idea. And I think everybody in every role it should reach out and experiment with this technology because it's going to become a fundamental [00:45:00] job skill for generating images even or, you know, PowerPoint or whatever else on those kinds of things.
Jim: Uh, it's gonna be a fundamental skill to keep up. From our standpoint, obviously, there's a lot of a lot of scrutiny that's going to need to occur within businesses as you do bring these products into your company and you know, you really need to come and understand what's coming down the pipe with that.
Jim: What's the cost of the business? What's fair use of these items? What's how can you protect your company? How can you protect your brand against these things? Uh, etcetera as well. Because injury to a brand is a problem as well. So, you know, if you look at the ModelOP solution, we help make this a lot easier, especially within large organizations.
Jim: So it's not painful to bring these in. It's not painful to become compliant, and you can find out more at ModelOP dot com. Uh, and, you know, you can find me on linked in under Jim Olsen under, uh, the But the model off banner as well. And I think you already have a link to it as well in [00:46:00] the bio. So please feel free to reach out and contact me with more questions about AI governance space and how it may apply to your company.
Jim: And we have a whole series of webcasts, blogs, etcetera on there as well on these topics, including industry leaders talking about how it's applied within their organization. So I encourage you to watch those as well.
Mehmet: Great. Thank you very much, Jim, again, for, you know, the time today and all the, you know, very valuable knowledge you shared with us.
Mehmet: And as you said, yes, so the audience, they don't have to look, you know, for the links. Uh, I will make the life easy putting them in the show notes. So if you're listening on your favorite podcasting app, you will find them in the show notes. If you're watching this on on youtube, you will find them in the description again.
Mehmet: Thank you very much, jim I really appreciate the time and all you know The the experience you shared with us and a very important topic which is ai governance because it touches also many important aspects from business perspective and also like [00:47:00] for us as humans as well because at the end of day You talked about the data, but it's our data whether it's like healthcare data financial data So thank you for bringing this here today.
Mehmet: And this is how I end my episode usually this is for the audience If you just discovered this podcast Thank you for passing by. I hope you enjoyed it. If you did, so please share it. And you know, uh, like, uh, on, on all our platforms that we broadcast on. And if you are one of the people who keep coming in, thank you for tuning in.
Mehmet: I appreciate all the support and your, you know, encouragement. And we'll meet again very soon in a new episode. Thank you. Bye bye.